Cracking the Code: Exploring Explainable AI (XAI)
Unleashing the Power of XAI: Unraveling the Mysteries of Explainable AI (XAI) Artificial Intelligence (AI) has taken the world by storm, redefining the way businesses operate and empowering them to base their strategies on data-driven insights.
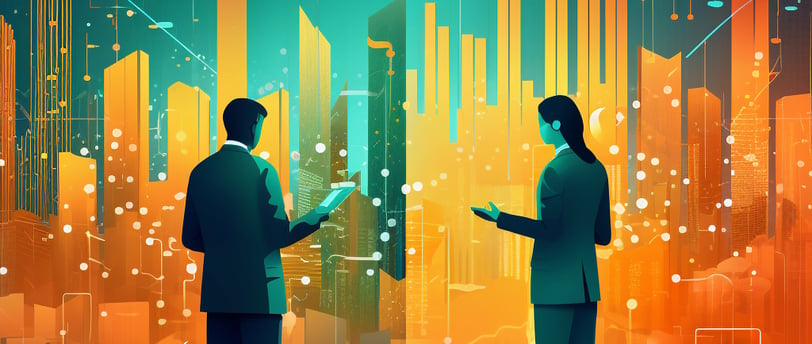
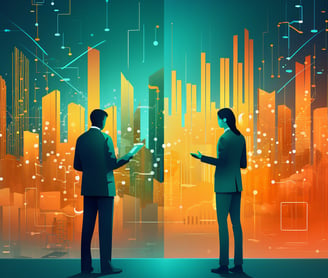
The rise of Artificial Intelligence has revolutionized numerous industries, empowering businesses to make informed decisions based on data. However, with the increasing complexity and sophistication of AI systems, it is crucial to understand how they arrive at their decisions. [1][2][3][4] That's where Explainable AI comes in – a rapidly developing field focused on creating transparent AI systems that provide clear explanations for their outputs and actions. [5][6][7] In this article, we will explore the concept of Explainable AI in depth, examining its key considerations and potential benefits for businesses. We will also discuss how Datasumi, a leading provider of AI solutions can assist companies in implementing Explainable AI technologies professionally.
Understanding Explainable AI (XAI)
Explainable AI (XAI) refers to the ability of AI systems to provide understandable explanations for their decisions and actions. Traditional AI models, such as deep neural networks, are often considered "black boxes" because they operate on complex algorithms that are difficult to interpret. This lack of interpretability raises concerns about bias, fairness, and trustworthiness, particularly critical in high-stakes domains such as healthcare, finance, and law. [8][9][5]
XAI aims to bridge this gap by making AI systems more transparent, interpretable, and accountable. It provides human-readable explanations for AI outputs, allowing users to understand the reasoning and logic behind the decisions. By uncovering the black box, XAI empowers businesses to gain insights into how AI systems arrive at conclusions, enabling them to validate, audit, and improve these systems effectively. [10][11][8][12]
Unpacking the Multifaceted Imperatives in Explainable Artificial Intelligence (XAI)
Artificial intelligence (AI) is transforming everything from healthcare to how these technologies reach their conclusions has become increasingly pressing. Organizations across various sectors are recognizing the need for transparency, fairness, and accountability in AI. [13][14][15][16][17] The concept of Explainable AI (XAI) serves as an answer to these critical concerns. XAI aims to make AI decision-making processes understandable and manageable, even for those without specialized technical knowledge. [18][19][5][20][21][22][23] The implications of failing to address these areas are substantial, ranging from legal repercussions to ethical violations and operational inefficiencies. Here are the critical concerns that organizations must consider in the realm of XAI:
Interpretability
The most pressing concern in the domain of XAI is interpretability—the capacity to understand and elucidate how an AI system arrives at specific conclusions or recommendations. For businesses to capitalize on AI technologies responsibly, they must grasp the underlying mechanisms that contribute to AI-generated outputs. Absent this understanding, there is a heightened risk of making pivotal decisions without full awareness of the potential biases and uncertainties in play. [24][21][12][6][25][26][27][28][29]
Fairness and Bias
AI algorithms can reinforce or even exacerbate existing societal biases if trained on skewed or unrepresentative data sets. Explainable AI sheds light on the variables influencing the AI's decisions, thus equipping businesses with the information needed to address such biases. This level of insight is crucial for upholding fairness and preventing inadvertent discrimination against particular groups, ultimately contributing to social equity.[30][31]
Trust and Accountability
The adage "trust, but verify" is particularly apt in the context of AI. Building trust in AI systems is essential for their broad acceptance and integration into operational workflows. XAI cultivates trust by offering clear, justifiable explanations for AI actions and decisions. This enhanced transparency equips organizations to hold their AI applications to account, thereby complying with legal and ethical standards. [32][33]
Robustness and Reliability
Consistency and performance are pivotal when deploying AI solutions in real-world settings. XAI strives to reveal vulnerabilities and weak spots in AI models, allowing organizations to pinpoint potential failure points and areas of uncertainty. Insight into how models behave under different circumstances enables organizations to fortify their AI systems, minimizing risks related to unexpected errors or failures. [34][35][36][37]
User-Centric Design
The effectiveness of AI technologies hinges not just on computational prowess but also on user engagement and experience. XAI focuses on designing intuitive AI interfaces and explanations tailored for non-technical audiences. By developing easy-to-understand visualizations and communication channels, XAI ensures that users can engage effectively with AI systems, facilitating the integration of AI insights into decision-making processes. [38][39][40][41][42][43]
Trade-off Between Performance and Explainability
The quest for higher accuracy in AI models often comes at the expense of explainability. More complex models may deliver better results but are typically harder to interpret. XAI works to balance these competing aspects, enabling organizations to select AI solutions that meet their unique requirements while maintaining a level of transparency and accountability. [44][45][46]
Scalability and Computational Complexity
As AI systems grow in complexity, the computational cost and time involved in explaining their decisions can become prohibitive. Research in XAI is directed towards creating efficient, scalable methods for detailing AI outputs, aiming to ensure that the need for interpretability doesn't hamper the adoption of more sophisticated AI technologies.[47][41]
Regulatory and Legal Compliance
The proliferation of AI technologies has prompted increased regulatory oversight. XAI provides a mechanism to demonstrate compliance with evolving laws concerning transparency and fairness. Clear, understandable explanations of AI decisions can facilitate regulatory assessments and help businesses stay within the boundaries of the law.[48][49][50][51]
Continuous Monitoring and Adaptation
Artificial intelligence models are dynamic and can change their decision-making processes over time. Explainable AI enables continuous monitoring and adaptation, helping organizations identify shifts. This ongoing oversight is crucial for ensuring that AI initiatives remain aligned with business objectives and ethical standards.[52][53]
Collaboration Between Experts
Developing effective and thorough Explainable AI solutions requires a multidisciplinary approach. It involves the collaboration of experts from various fields such as AI researchers, ethicists, psychologists, and industry professionals. By working together in an interdisciplinary fashion, these professionals can create more comprehensive XAI frameworks that tackle the complex issues surrounding interpretability, fairness, and accountability.[54][55][18][5][56]
The critical concerns in Explainable AI encompass a wide array of considerations—from interpretability and fairness to trust and robustness, among others. Addressing these multi-dimensional aspects is fundamental to unleashing the full potential of AI technologies responsibly. As AI continues to infiltrate various sectors, the imperatives of XAI will remain central to ethical and effective deployment.
Unlocking Business Value Through Explainable AI (XAI)
Artificial Intelligence (AI) has become a cornerstone for innovation and efficiency. However, the 'black box' nature of many AI algorithms has raised concerns regarding transparency, reliability, and ethical considerations. Enter Explainable AI (XAI), a game-changing paradigm that not only offers intelligent solutions but also makes the decision-making process of these systems interpretable and transparent. The following explores the manifold benefits that XAI brings to businesses, substantiating why it is an essential component for modern organizations.[57][4][58]
Comprehensive Enhancement in Decision-Making
XAI serves as a critical tool for improving decision-making capabilities within organizations. It goes beyond merely delivering outputs and provides actionable insights that elucidate how AI systems arrive at specific conclusions. By dissecting and understanding the factors that influence AI decisions, businesses across various sectors—be it finance, healthcare, marketing, or supply chain management—can make data-driven choices with a higher degree of confidence. This level of clarity can help in validating AI recommendations, identifying possible errors or biases, and ultimately bolstering overall decision-making efficacy.[59][60][61][62][63]
Meeting Regulatory and Ethical Standards
In an era where regulatory bodies are increasingly focusing on data privacy and ethical concerns, XAI stands out as an enabler for compliance. It provides transparent explanations for how AI algorithms produce specific outputs, allowing businesses to demonstrate the fairness and accountability of their AI systems. Adopting XAI techniques effectively mitigates the risks associated with legal repercussions and non-compliance, ensuring that organizations meet the standards set by regulatory agencies.[4][64][65][66]
Elevating User Experience to New Heights
XAI enhances user experience by offering explanations that demystify AI-driven recommendations or predictions. Take the example of an e-commerce platform: XAI can reveal the underlying factors that influence product recommendations. This transparency increases user trust and fosters satisfaction, leading to higher customer retention rates. Similarly, in healthcare, XAI can provide evidence-based justifications for diagnosis or treatment suggestions, thereby empowering both medical practitioners and patients to make more informed decisions.[67][68]
Gaining Competitive Advantage Through Transparency
As the landscape becomes increasingly crowded, the ability to offer something unique becomes a defining competitive edge. Businesses that proactively adopt XAI set themselves apart by delivering AI solutions that are not just effective but also transparent and easily interpretable. This level of transparency can serve as a unique selling point, building greater trust among customers. Over time, this can enhance brand reputation, solidify customer loyalty, and pave the way for long-term business success.[69][70]
Explainable AI is not just an optional add-on; it is quickly becoming a necessity for businesses aiming to be both ethical and competitive. By facilitating better decision-making, ensuring compliance, improving user experience, and offering a competitive advantage, XAI can be a catalyst for business transformation and growth.
Navigating the Complex Landscape of Explainable AI: Key Principles for Success
Explainable AI (XAI) has become a linchpin for organizations striving for transparency, accountability, and ethical compliance in their AI-driven processes. As AI systems continue to become more complex and deeply integrated into various sectors, the importance of making these systems interpretable cannot be overstated. For businesses aiming to succeed in deploying XAI solutions, here are some critical insights that serve as pillars for developing trustworthy, understandable, and responsible AI systems.[19][71][45]
Key Pillars for Success in XAI
Domain Expertise
Achieving success in XAI mandates a profound comprehension of both AI methodologies and the particular industry where the AI solution will be deployed. Companies should look for collaborative opportunities with domain experts who possess the requisite knowledge to design interpretable models that are compliant with industry standards and regulations.[72][73][74][75][19]
Data Quality and Bias Mitigation
High-quality, representative, and unbiased training data is the bedrock upon which reliable and fair AI systems are built. Organizations need to curate diverse datasets and invest in robust preprocessing techniques that can identify and neutralize biases. This ensures that AI systems make equitable and unbiased decisions, aligning with the goals of XAI.[76][77][78][79]
Model Selection and Design
It's crucial to understand that not all AI models offer the same level of interpretability. Businesses must judiciously choose or design models that strike a balance between performance and explainability. Employing techniques like decision trees, rule-based systems, and model-agnostic methods can make AI systems more interpretable without compromising on accuracy.[5][80][81][82][83][84]
Post Hoc Explanations
In situations where complex, 'black-box' AI models are already in use, post hoc explanation techniques come into play. These methods aim to elucidate the outputs of such models by approximating their functionality or dissecting their internal mechanics. Organizations must incorporate these explanation techniques to better comprehend their existing AI systems' decision-making processes.[85][86][87][88][89][90]
Transparency and Documentation
Transparent documentation is a cornerstone of responsible AI. Complete and understandable documentation of AI model architectures, data preparation, and decision-making algorithms allows stakeholders, including regulatory bodies and end-users, to fully grasp the AI decision-making process. This level of transparency fosters trust and simplifies the resolution of potential issues.[91][92][46]
Human-AI Collaboration
XAI champions the integration of human expertise into the decision-making loop. Companies should prioritize developing AI solutions that seamlessly collaborate with humans, including the design of intuitive interfaces, real-time explanations during decision-making, and options for human override. Such human-in-the-loop systems make AI more adaptable and attuned to dynamic situations.[93][94][95][96][97]
Ethical Considerations
A robust ethical framework should underlie all aspects of XAI, from the development to the deployment stages. Organizations need to be conscious of the ethical implications of their AI decisions and provide explanations that are mindful of privacy, fairness, and societal norms. Establishing ethical guidelines for XAI aids in responsibly navigating the moral complexities of AI technology.[98][99]
Continuous Monitoring and Improvement
The journey of XAI does not conclude with the deployment of the AI model; it's an ongoing commitment. Companies should set up procedures for the continual monitoring of performance, explanations, and societal impact. Periodic assessments facilitate prompt adjustments, ensuring the AI systems remain aligned with their intended objectives.[100][101]
User-Centric Evaluation
For XAI to be truly effective, the end-user's perspective should be the primary evaluation metric. Gathering user feedback on the clarity and utility of the explanations allows for iterative improvements. This ensures that XAI solutions are genuinely beneficial to the target audience.[102][103][104]
Education and Training
Empowering the workforce with an understanding of AI, interpretability techniques, and ethical considerations is essential. Educated employees are more adept at leveraging AI insights and can identify potential pitfalls such as biases or inaccuracies.[105]
Collaboration and Knowledge Sharing
The realm of XAI is ever-evolving. Staying abreast of emerging trends and best practices requires active collaboration and knowledge exchange within the AI community. Engaging in specialized conferences, workshops, and forums allows organizations to both learn from and contribute to the broader discourse on interpretable AI.[106][97][107]
By adhering to these guiding principles, businesses can successfully navigate the complexities of XAI and unlock the vast potential of AI systems that are both interpretable and ethically responsible.
Conclusion
Explainable AI (XAI) is a critical aspect of AI adoption for businesses. By enabling transparent and interpretable AI systems, XAI empowers organizations to make informed decisions, address biases, build trust, and gain a competitive advantage. Understanding the key concerns, potential benefits, and crucial insights for success in XAI is essential for businesses seeking to leverage this technology effectively. Datasumi, with its expertise in XAI, can be a valuable partner in helping businesses crack the code of Explainable AI and drive their success in an increasingly AI-driven world.
At its core, XAI is about demystifying the decision-making processes of AI systems, granting organizations the ability to grasp how and why specific outcomes are produced. This interpretability is a game-changer, as it empowers businesses to make well-informed decisions that are not shrouded in ambiguity. This newfound clarity transcends industries, from finance and healthcare to manufacturing and marketing, enabling professionals to confidently rely on AI insights for critical choices that drive their operations forward.
One of the most compelling advantages XAI offers is the ability to address biases inherent in AI systems. Biases, often unintentionally ingrained during the training phase, can lead to unfair and unjust outcomes. With its focus on transparency, XAI provides a means to identify, understand, and rectify these biases. By shining a light on the decision-making process, XAI equips businesses with the tools to mitigate biases, ensure fairness, and promote inclusivity – a profound step toward a more just and equitable AI landscape.
Trust, a cornerstone of any successful business relationship, is significantly bolstered through XAI. Building trust is paramount in an era where AI's influence on decision-making is increasing. XAI fosters this trust by offering clear explanations for AI decisions, allowing stakeholders to verify the validity and rationale behind each outcome. Organizations can demonstrate their commitment to ethical AI practices, regulatory compliance, and societal expectations, cultivating a reputation for responsible and accountable AI usage.
Beyond the ethical imperative, the benefits of XAI translate into a competitive edge. Businesses that harness the power of interpretable AI systems position themselves as innovators and pioneers in their respective fields. They not only gain insights that drive efficiency and optimize operations but also differentiate themselves by showcasing a commitment to cutting-edge technology that is understandable and controllable.
the complex realm of XAI, businesses should possess essential knowledge and insights for success. These insights cover various aspects including domain expertise, data quality, model selection, and user-centric design. Datasumi emerges as a trusted source of expertise and guidance on this journey. As a leading AI company, Datasumi offers specialized services such as XAI consulting, model development, bias detection, post-hoc explanations,user-centric interface design, and ethical frameworks. By providing comprehensive support Datasumi supports businesses to strategic assistance required to unlock the potential of XAI.
In a world increasingly shaped by AI, organizations that embrace XAI with the guidance of Datasumi are poised to seize a remarkable opportunity. By blending the power of AI insights with transparency, accountability, and ethical responsibility, businesses can forge a path toward success in an AI-driven landscape where understanding and collaboration define the future.
References
Artificial Intelligence and Employment - OECD. https://www.oecd.org/future-of-work/reports-and-data/AI-Employment-brief-2021.pdf.
The Rise Of AI (Artificial Intelligence) In The Workplace - Forbes. https://www.forbes.com/sites/forbesbooksauthors/2023/05/18/the-rise-of-ai-in-the-workplace/.
The Rise of Artificial Intelligence (AI) in Business: Opportunities .... https://nnroad.com/blog/rise-of-ai-in-business/.
Ethical concerns mount as AI takes bigger decision-making role. https://news.harvard.edu/gazette/story/2020/10/ethical-concerns-mount-as-ai-takes-bigger-decision-making-role/.
What is explainable AI? | IBM. https://www.ibm.com/topics/explainable-ai.
Explainable AI: A Review of Machine Learning Interpretability Methods. https://www.ncbi.nlm.nih.gov/pmc/articles/PMC7824368/.
Explainable AI | Google Cloud. https://cloud.google.com/explainable-ai/.
Explainable artificial intelligence - Wikipedia. https://en.wikipedia.org/wiki/Explainable_artificial_intelligence.
What is Explainable AI (XAI)? | Definition from WhatIs - TechTarget. https://www.techtarget.com/whatis/definition/explainable-AI-XAI.
Explainable AI (XAI): Core Ideas, Techniques, and Solutions. https://dl.acm.org/doi/10.1145/3561048.
Adopting XAI to Build Transparent and Accountable AI Systems. https://inapp.com/blog/adopting-xai-to-build-transparent-and-accountable-ai-systems/.
XAIR: A Systematic Metareview of Explainable AI (XAI) Aligned to the .... https://www.mdpi.com/2504-4990/5/1/6.
Transforming healthcare with AI: The impact on the workforce and .... https://www.mckinsey.com/industries/healthcare/our-insights/transforming-healthcare-with-ai.
Artificial intelligence is transforming our world — it is on all of us .... https://ourworldindata.org/ai-impact.
How AI is Transforming the Future of Healthcare - International SOS. https://www.internationalsos.com/magazine/how-ai-is-transforming-the-future-of-healthcare.
Artificial intelligence (AI) | Definition, Examples, Types .... https://www.britannica.com/technology/artificial-intelligence.
Artificial Intelligence: Transforming Healthcare, Cybersecurity, and .... https://www.forbes.com/sites/chuckbrooks/2023/09/04/artificial-intelligence-transforming-healthcare-cybersecurity-and-communications/.
Explainable for Trustworthy AI | SpringerLink. https://link.springer.com/chapter/10.1007/978-3-031-24349-3_10.
XAI—Explainable artificial intelligence | Science Robotics. https://www.science.org/doi/10.1126/scirobotics.aay7120.
What Is Explainable AI (XAI)? | NVIDIA Blog. https://blogs.nvidia.com/blog/2021/05/24/what-is-explainable-ai/.
Explainable Artificial Intelligence (XAI): What we know and what is .... https://www.sciencedirect.com/science/article/pii/S1566253523001148.
Explainable AI (XAI): Explained | IEEE Conference Publication - IEEE Xplore. https://ieeexplore.ieee.org/document/10134984/.
XAI—Explainable artificial intelligence - Science | AAAS. https://www.science.org/doi/pdf/10.1126/scirobotics.aay7120.
Explainable digital forensics AI: Towards mitigating distrust in AI .... https://www.sciencedirect.com/science/article/pii/S2666281722000841.
Explainable AI in radiology: a white paper of the Italian ... - Springer. https://link.springer.com/article/10.1007/s11547-023-01634-5.
Notions of explainability and evaluation approaches for explainable .... https://www.sciencedirect.com/science/article/pii/S1566253521001093.
A comprehensive taxonomy for explainable artificial intelligence: a .... https://link.springer.com/article/10.1007/s10618-022-00867-8.
Coarse ethics: how to ethically assess explainable artificial .... https://link.springer.com/article/10.1007/s43681-021-00091-y.
Explainable Artificial Intelligence (XAI): Concepts, Taxonomies .... https://www.researchgate.net/publication/338184751_Explainable_Artificial_Intelligence_XAI_Concepts_Taxonomies_Opportunities_and_Challenges_toward_Responsible_AI.
Tackling bias in artificial intelligence (and in humans) | McKinsey. https://www.mckinsey.com/featured-insights/artificial-intelligence/tackling-bias-in-artificial-intelligence-and-in-humans.
AI bias: exploring discriminatory algorithmic decision ... - Springer. https://link.springer.com/article/10.1007/s43681-022-00138-8.
Trust, but verify: The quest to measure our trust in AI. https://news.asu.edu/20230207-trust-verify-quest-measure-our-trust-ai.
Artificial Intelligence’s Biggest Stumbling Block: Trust - Forbes. https://www.forbes.com/sites/joemckendrick/2021/08/30/artificial-intelligences-biggest-stumbling-block-trust/.
Building AI Vision Systems in the Real World | insight.tech. https://www.insight.tech/content/building-ai-vision-systems-in-the-real-world.
3 requirements for successful artificial intelligence programs. https://mitsloan.mit.edu/ideas-made-to-matter/3-requirements-successful-artificial-intelligence-programs.
Building the AI-Powered Organization - Harvard Business Review. https://hbr.org/2019/07/building-the-ai-powered-organization.
National AI Engineering Initiative Robust and Secure AI. https://resources.sei.cmu.edu/asset_files/WhitePaper/2021_019_001_735346.pdf.
Artificial Intelligence (AI): Multidisciplinary perspectives on .... https://www.sciencedirect.com/science/article/pii/S026840121930917X.
Managing AI Decision-Making Tools - Harvard Business Review. https://hbr.org/2021/11/managing-ai-decision-making-tools.
AI Isn’t Ready to Make Unsupervised Decisions - Harvard Business Review. https://hbr.org/2022/09/ai-isnt-ready-to-make-unsupervised-decisions.
How artificial intelligence is transforming the world | Brookings. https://www.brookings.edu/articles/how-artificial-intelligence-is-transforming-the-world/.
The promise and challenges of AI - American Psychological Association (APA). https://www.apa.org/monitor/2021/11/cover-artificial-intelligence.
The present and future of AI - Harvard John A. Paulson School of .... https://seas.harvard.edu/news/2021/10/present-and-future-ai.
How to Build Accountability into Your AI - Harvard Business Review. https://hbr.org/2021/08/how-to-build-accountability-into-your-ai.
Explainable artificial intelligence (XAI) in banking | Deloitte Insights. https://www2.deloitte.com/us/en/insights/industry/financial-services/explainable-ai-in-banking.html.
AI transparency: What is it and why do we need it? | TechTarget. https://www.techtarget.com/searchcio/tip/AI-transparency-What-is-it-and-why-do-we-need-it.
The computing power needed to train AI is now rising seven times faster .... https://www.technologyreview.com/2019/11/11/132004/the-computing-power-needed-to-train-ai-is-now-rising-seven-times-faster-than-ever-before/.
The AI Transparency Paradox - Harvard Business Review. https://hbr.org/2019/12/the-ai-transparency-paradox.
The EU and U.S. are starting to align on AI regulation. https://www.brookings.edu/articles/the-eu-and-u-s-are-starting-to-align-on-ai-regulation/.
AI Regulation Is Coming - Harvard Business Review. https://hbr.org/2021/09/ai-regulation-is-coming.
Ten Ways AI Regulations and Standards Will Evolve in 2022. https://innovationatwork.ieee.org/ten-ways-ai-regulations-and-standards-will-evolve-in-2022/.
Explainable AI: Getting it right in business | McKinsey. https://www.mckinsey.com/capabilities/quantumblack/our-insights/why-businesses-need-explainable-ai-and-how-to-deliver-it.
How Artificial Intelligence Can Improve Organizational Decision Making. https://www.forbes.com/sites/forbestechcouncil/2022/08/23/how-artificial-intelligence-can-improve-organizational-decision-making/.
The perils and pitfalls of explainable AI: Strategies for explaining .... https://www.sciencedirect.com/science/article/pii/S0740624X21001027.
Explainability for artificial intelligence in healthcare: a .... https://www.ncbi.nlm.nih.gov/pmc/articles/PMC7706019/.
Explainable Artificial Intelligence in Data Science. https://link.springer.com/article/10.1007/s11023-022-09603-z.
The transformative potential of artificial intelligence. https://www.sciencedirect.com/science/article/pii/S0016328721001932.
SQ2. What are the most important advances in AI?. https://ai100.stanford.edu/gathering-strength-gathering-storms-one-hundred-year-study-artificial-intelligence-ai100-2021-1/sq2.
What Are People Doing About XAI User Experience? A Survey on AI .... https://link.springer.com/chapter/10.1007/978-3-030-49760-6_4.
Explainable AI (XAI): A systematic meta-survey of current challenges .... https://www.sciencedirect.com/science/article/pii/S0950705123000230.
Explainable Artificial Intelligence (XAI) techniques for energy and .... https://www.sciencedirect.com/science/article/pii/S2666546822000246.
Current Challenges and Future Opportunities for XAI in Machine ... - MDPI. https://www.mdpi.com/2076-3417/11/11/5088.
Advances in XAI: Explanation Interfaces in Healthcare. https://link.springer.com/chapter/10.1007/978-3-030-83620-7_15.
Data and Cybersecurity: 2023 Regulatory Challenges - KPMG. https://advisory.kpmg.us/articles/2022/ten-key-regulatory-challenges-2023-data-cybersecurity.html.
Privacy and artificial intelligence: challenges for protecting health .... https://bmcmedethics.biomedcentral.com/articles/10.1186/s12910-021-00687-3.
Ethical data usage in an era of digital technology and regulation. https://www.mckinsey.com/capabilities/mckinsey-digital/our-insights/tech-forward/ethical-data-usage-in-an-era-of-digital-technology-and-regulation.
Explainable Artificial Intelligence (XAI): Understanding and Future .... https://link.springer.com/chapter/10.1007/978-3-031-18292-1_2.
Who needs explanation and when? Juggling explainable AI and user .... https://www.sciencedirect.com/science/article/pii/S1071581922000660.
Competitive edge | Product positioning in your target market. https://learn.marsdd.com/article/defining-your-competitive-edge/.
5.3 Competitive Analysis - Entrepreneurship | OpenStax. https://openstax.org/books/entrepreneurship/pages/5-3-competitive-analysis.
Explainable AI, But Explainable to Whom? An Exploratory Case ... - Springer. https://link.springer.com/chapter/10.1007/978-3-030-83620-7_7.
A review of Explainable AI (XAI) concepts, techniques, and challenges. https://www2.deloitte.com/uk/en/pages/deloitte-analytics/articles/a-review-of-explainable-ai-concepts-techniques-and-challenges.html.
Explainable Artificial Intelligence in education - ScienceDirect. https://www.sciencedirect.com/science/article/pii/S2666920X22000297.
XAI Systems Evaluation: A Review of Human and Computer-Centred ... - MDPI. https://www.mdpi.com/2076-3417/12/19/9423/htm.
Explainability won’t save AI | Brookings. https://www.brookings.edu/articles/explainability-wont-save-ai/.
Training Data: What Is It? All About Machine Learning Training Data - Appen. https://appen.com/blog/training-data/.
Today’s AI Solutions Require Quality, Unbiased Training Data - Forbes. https://www.forbes.com/sites/forbesbusinesscouncil/2020/08/26/todays-ai-solutions-require-quality-unbiased-training-data/.
What Do We Do About the Biases in AI? - Harvard Business Review. https://hbr.org/2019/10/what-do-we-do-about-the-biases-in-ai.
Training Data Quality: Why It Matters in Machine Learning. https://www.v7labs.com/blog/quality-training-data-for-machine-learning-guide.
AI Can Be Both Accurate and Transparent - Harvard Business Review. https://hbr.org/2023/05/ai-can-be-both-accurate-and-transparent.
What is Interpretability - Interpretable AI. https://www.interpretable.ai/interpretability/what/.
The Ultimate Guide to Understanding and Using AI Models (2023) - Viso. https://viso.ai/deep-learning/ml-ai-models/.
When — and Why — You Should Explain How Your AI Works. https://hbr.org/2022/08/when-and-why-you-should-explain-how-your-ai-works.
6 – Interpretability – Machine Learning Blog - ML@CMU. https://blog.ml.cmu.edu/2020/08/31/6-interpretability/.
Why Are We Using Black Box Models in AI When We Don’t Need To? A Lesson .... https://hdsr.mitpress.mit.edu/pub/f9kuryi8.
The false hope of current approaches to explainable artificial .... https://www.thelancet.com/journals/landig/article/PIIS2589-7500(21)00208-9/fulltext.
Managing the black box of artificial intelligence (AI) - Deloitte US. https://www2.deloitte.com/us/en/pages/advisory/articles/black-box-artificial-intelligence.html.
Transparency and the Black Box Problem: Why We Do Not Trust AI - Springer. https://link.springer.com/article/10.1007/s13347-021-00477-0.
Stop explaining black box machine learning models for high stakes .... https://www.nature.com/articles/s42256-019-0048-x.
Explainable artificial intelligence (XAI) post-hoc explainability .... https://link.springer.com/article/10.1007/s43681-022-00142-y.
Transparency is key to responsible AI - Deloitte US. https://www2.deloitte.com/dk/da/pages/technology/articles/transparency-is-key-to-responsible.html.
Transparency and explainability (OECD AI Principle) - OECD.AI. https://oecd.ai/en/dashboards/ai-principles/P7.
The human-AI relationship in decision-making - arXiv.org. https://arxiv.org/pdf/2102.05460.
Human-centered XAI: Developing design patterns for explanations of clinical decision support systems. https://www.sciencedirect.com/science/article/pii/S1071581921001026.
Explainable Artificial Intelligence (XAI): Connecting Artificial .... https://link.springer.com/chapter/10.1007/978-981-19-1142-2_10.
(PDF) The Impact of Imperfect XAI on Human-AI Decision-Making. https://www.researchgate.net/publication/372625783_The_Impact_of_Imperfect_XAI_on_Human-AI_Decision-Making.
Explainable Artificial Intelligence - DARPA. https://www.darpa.mil/program/explainable-artificial-intelligence.
From ethical AI frameworks to tools: a review of approaches. https://link.springer.com/article/10.1007/s43681-023-00258-9.
The Ethics of AI Ethics: An Evaluation of Guidelines. https://link.springer.com/article/10.1007/s11023-020-09517-8.
Unbox the black-box for the medical explainable AI via multi-modal and .... https://www.ncbi.nlm.nih.gov/pmc/articles/PMC8459787/.
Explainable Artificial Intelligence (XAI) (Archived) - DARPA. https://www.darpa.mil/program/explainable-artificial-intelligence.
How to explain AI systems to end users: a systematic literature review .... https://www.emerald.com/insight/content/doi/10.1108/INTR-08-2021-0600/full/html.
Explainable Artificial Intelligence (XAI) from a user perspective: A .... https://www.sciencedirect.com/science/article/pii/S0040162522006412.
IEEE JOURNAL, VOL. XX, NO. X, DECEMBER 2021 1 Explainable Artificial .... https://arxiv.org/pdf/2112.12705.pdf.
Realizing the full potential of AI in the workplace | Deloitte Insights. https://www2.deloitte.com/us/en/insights/focus/technology-and-the-future-of-work/ai-in-the-workplace.html.
XAI—Explainable artificial intelligence | Science Robotics - AAAS. https://www.science.org/doi/10.1126/scirobotics.aay7120.
The 5 Biggest Artificial Intelligence (AI) Trends In 2023 - Forbes. https://www.forbes.com/sites/bernardmarr/2022/10/10/the-5-biggest-artificial-intelligence-ai-trends-in-2023/.