Real-Time Data Analytics Helps Predicting Demand
7/21/20248 min read
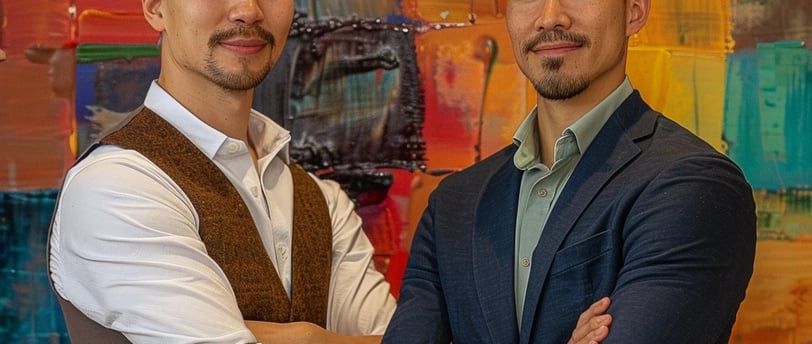
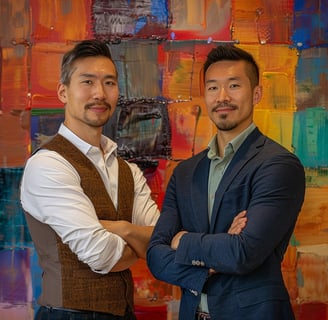
In today's fast-paced and ever-changing market landscape, accurately predicting demand is crucial for businesses to remain competitive and responsive to consumer needs. Traditional demand forecasting methods, which rely heavily on historical data and periodic updates, often fall short in providing the precision and agility required. These conventional approaches can lead to significant gaps between forecasted and actual demand, resulting in either excess inventory or stockouts, both of which can have adverse financial impacts.
Real-time data analytics emerges as a transformative solution in this context, offering a more dynamic and responsive approach to demand forecasting. By continuously collecting and analyzing data as it is generated, real-time data analytics enables businesses to capture the most current market trends and consumer behaviors. This immediacy in data processing allows for more accurate demand predictions, helping companies adjust their strategies promptly to align with real-time market conditions.
Moreover, real-time data analytics leverages advanced technologies such as machine learning, artificial intelligence, and big data to process vast amounts of information from various sources. These sources can include social media, online transactions, IoT devices, and other digital touchpoints, providing a comprehensive view of the market environment. As a result, businesses can identify patterns and anomalies that might be missed with traditional methods, leading to more informed and effective decision-making.
In essence, the integration of real-time data analytics into demand forecasting represents a significant advancement, enabling organizations to anticipate demand fluctuations with greater accuracy. This proactive approach not only enhances inventory management but also improves customer satisfaction by ensuring that products are available when and where they are needed. As we delve deeper into this blog post, we will explore the various facets of real-time data analytics and its profound impact on demand forecasting.
Capturing Current Demand Signals
Real-time data analytics plays a pivotal role in capturing immediate visibility into current demand patterns, offering businesses the agility to respond swiftly and accurately. By continuously monitoring various data streams, companies can identify sudden changes in consumer behavior, emerging trends, and seasonal variations. This instantaneous insight is crucial for adapting to dynamic market conditions and staying ahead of the competition.
One of the key advantages of real-time data is the ability to detect and analyze sudden spikes or drops in demand. For example, if a particular product experiences an unexpected surge in popularity, businesses can quickly adjust their inventory levels, marketing strategies, and production schedules to meet the increased demand. Conversely, if demand for a product declines unexpectedly, companies can take proactive measures to avoid overstocking and potential financial losses.
Real-time data also helps in identifying emerging trends that may not yet be evident through traditional data collection methods. By analyzing social media activity, online searches, and other digital interactions, businesses can gain early insights into shifting consumer preferences and adjust their offerings accordingly. This proactive approach allows companies to capitalize on new opportunities and stay relevant in an ever-changing marketplace.
Seasonal variations in demand are another critical factor that can be effectively managed with real-time data analytics. By continuously monitoring sales patterns and external factors such as weather conditions or cultural events, businesses can anticipate and prepare for fluctuations in demand. This ensures that they are well-equipped to handle peak periods and maintain optimal stock levels throughout the year.
Integrating real-time data into demand forecasting models is essential for improving the accuracy of future predictions. Traditional forecasting methods often rely on historical data, which may not adequately reflect current market conditions. By incorporating real-time data, businesses can create more responsive and adaptive forecasting models, leading to better decision-making and enhanced operational efficiency.
Enhanced Granularity and Detail
Real-time data analytics empowers organizations with a higher level of granularity and detail in their data analysis processes. This granularity is essential for understanding demand at a more intricate level, which traditional data analysis methods often fail to capture. Enhanced granularity enables businesses to dissect their data into smaller, more specific segments, allowing them to identify micro-trends that might otherwise go unnoticed. These micro-trends can provide valuable insights into consumer behaviors and preferences, driving more precise demand forecasting.
One of the key advantages of real-time analytics is its ability to highlight regional preferences. By analyzing data in real-time, businesses can observe how demand fluctuates across different geographical areas. This localized insight is crucial for tailoring marketing strategies, inventory management, and distribution plans to meet the unique needs of each region. For example, a product that is popular in urban areas may not have the same level of demand in rural regions. Understanding these regional preferences can help organizations optimize their supply chain and improve customer satisfaction.
Moreover, real-time data analytics enables companies to delve into specific customer behaviors. By monitoring consumer interactions and transactions as they happen, businesses can gain a deeper understanding of purchasing patterns, preferences, and triggers. This detailed level of insight allows for more targeted marketing efforts and the development of personalized offerings that resonate with individual customers. For instance, if a retailer notices a spike in demand for a particular product category during a specific time of year, they can proactively adjust their inventory and marketing campaigns to capitalize on this trend.
In essence, the enhanced granularity and detail provided by real-time data analytics are instrumental in refining demand forecasting. By identifying micro-trends, regional preferences, and specific customer behaviors, organizations can make more informed decisions, reduce the risk of overstocking or understocking, and ultimately, drive better business outcomes.
Improved Responsiveness to Market Changes
Real-time data analytics has revolutionized the way businesses respond to ever-changing market conditions. By providing immediate insights into market trends and consumer behavior, organizations can make swift and informed decisions to adjust their inventory, pricing, and marketing strategies. This agility allows companies to stay competitive and meet customer demands more effectively.
One of the critical advantages of real-time data analytics is the ability to monitor and analyze market conditions as they evolve. For instance, if a sudden surge in demand for a particular product is detected, businesses can promptly increase inventory levels to prevent stockouts. Conversely, if a decline in demand is observed, companies can reduce inventory to avoid overstocking and minimize holding costs. This real-time adjustment capability optimizes resource allocation and enhances operational efficiency.
Pricing strategies also benefit significantly from real-time data analytics. Dynamic pricing models, powered by real-time data, allow businesses to adjust prices based on current market conditions, competitor pricing, and consumer purchasing patterns. For example, e-commerce platforms often use real-time analytics to offer personalized discounts and promotions to customers, thereby boosting sales and customer satisfaction.
Marketing strategies can be fine-tuned using real-time insights as well. By analyzing consumer behavior data, businesses can identify emerging trends and preferences, enabling them to tailor marketing campaigns to target specific customer segments more effectively. This targeted approach not only improves the return on investment for marketing efforts but also enhances customer engagement and loyalty.
Industries such as retail, e-commerce, and manufacturing have seen significant improvements in demand prediction and operational efficiency due to real-time data analytics. For example, retail giants like Amazon utilize real-time data to optimize their supply chain and inventory management, ensuring products are available when and where customers need them. Similarly, manufacturers use real-time analytics to streamline production processes and reduce lead times, ultimately improving product availability and customer satisfaction.
Integration with Advanced Technologies
Real-time data analytics, when integrated with advanced technologies like machine learning (ML), artificial intelligence (AI), and the Internet of Things (IoT), becomes a potent tool for predicting demand with enhanced accuracy. The synergy between these technologies allows organizations to harness a broader spectrum of data points and apply more sophisticated modeling techniques, thereby refining the predictive capabilities of their analytics systems.
Machine learning algorithms can process vast amounts of data in real time, identifying patterns and trends that may not be immediately apparent through traditional analytical methods. By continuously learning from new data, ML models can adapt to changing market conditions, ensuring that demand forecasts remain relevant and precise. This adaptability is particularly advantageous in industries with volatile demand patterns, where traditional static models might fall short.
Artificial intelligence further augments the predictive power of real-time data analytics by enabling the creation of complex models that can simulate various scenarios. AI-driven predictive analytics can consider a multitude of variables simultaneously, providing a more comprehensive understanding of potential demand fluctuations. For example, AI can analyze consumer behavior, economic indicators, and seasonal trends to predict future demand more accurately, allowing businesses to make informed decisions about inventory management and resource allocation.
The integration of IoT devices adds another layer of data richness to real-time analytics. IoT sensors and devices generate continuous streams of data from various sources, such as weather conditions, supply chain logistics, and in-store customer interactions. By incorporating this real-time IoT data into predictive models, businesses can gain insights into external factors that influence demand, leading to more dynamic and responsive forecasting. For instance, a retailer could adjust stock levels based on real-time foot traffic data, ensuring optimal inventory at all times.
Overall, the amalgamation of real-time data analytics with advanced technologies such as ML, AI, and IoT provides a robust framework for more accurate and dynamic demand forecasting. These integrations not only enhance the predictive power of analytics but also empower businesses to respond proactively to market changes, ultimately driving efficiency and competitiveness.
Case Studies and Real-World Applications
Real-time data analytics has proven to be a game-changer in various industries by significantly enhancing demand forecasting accuracy. One notable example is the retail giant Walmart. By leveraging real-time data analytics, Walmart has been able to predict customer demand with remarkable precision. The company utilized a combination of historical sales data, weather patterns, and social media trends to forecast demand for various products. As a result, Walmart improved its inventory management, reducing stockouts and overstock situations, which led to increased customer satisfaction and operational efficiency.
In the manufacturing sector, Toyota has successfully implemented real-time data analytics to streamline its supply chain management. By analyzing data from production lines, supplier networks, and market demand in real-time, Toyota can adjust its manufacturing processes dynamically. This has led to a significant reduction in lead times, minimized production delays, and optimized resource allocation. The improved demand prediction accuracy has also enabled Toyota to align its production schedules more closely with market needs, enhancing overall operational efficiency.
The airline industry also benefits from real-time data analytics. For instance, Delta Air Lines uses real-time data to monitor passenger booking patterns, weather forecasts, and flight status updates. By analyzing this data, Delta can predict flight demand more accurately, optimize flight schedules, and allocate resources more effectively. This has resulted in better on-time performance, reduced operational costs, and improved customer satisfaction.
Another compelling case is Amazon, which employs real-time data analytics to anticipate customer purchase behaviors. By analyzing browsing patterns, purchase history, and demographic data, Amazon can predict which products are likely to be in high demand. This enables the company to manage its inventory more efficiently, ensuring that popular items are readily available for quick shipping. Consequently, Amazon has seen significant improvements in customer satisfaction and loyalty.
These examples illustrate the broad applicability and substantial benefits of real-time data analytics in predicting demand across different industries. Businesses that harness the power of real-time data can achieve greater accuracy in demand forecasting, enhance operational efficiency, and ultimately improve customer satisfaction.
Conclusion and Future Trends
In the rapidly evolving landscape of demand forecasting, real-time data analytics has emerged as a game-changer. Throughout this discussion, we have highlighted how real-time analytics aids in making more accurate predictions, enhancing decision-making processes, and optimizing inventory management. By leveraging real-time data, businesses can respond swiftly to market changes, reducing the risk of overstocking or stockouts, and ultimately improving customer satisfaction.
The transformative impact of real-time data analytics is evident across various industries. As companies continue to harness the power of real-time data, we can expect several future trends to shape this field further. One significant development is the advancement in data processing technologies. Innovations in cloud computing and edge computing are making it possible to handle vast amounts of data more efficiently, ensuring that real-time analytics can be performed seamlessly.
Additionally, the broader adoption of Artificial Intelligence (AI) and Machine Learning (ML) promises to elevate real-time data analytics to new heights. AI-powered algorithms can analyze data patterns more accurately and predict demand with greater precision. These technologies can also automate many aspects of the analytics process, reducing the need for manual intervention and allowing businesses to focus on strategic decision-making.
Moreover, as the market environment becomes increasingly fast-paced, the importance of real-time analytics will only grow. Businesses will need to stay agile and responsive to ever-changing consumer preferences and market conditions. Real-time data analytics will be crucial in providing the insights necessary to adapt quickly and maintain a competitive edge.
In conclusion, real-time data analytics is not just a tool but a strategic asset that can significantly enhance demand forecasting capabilities. As technology continues to evolve, the future of real-time analytics looks promising, with advancements that will drive even more accurate and timely insights, helping businesses thrive in a dynamic marketplace.