Retrieval-Augmented Generation (RAG): The Future of Generative AI
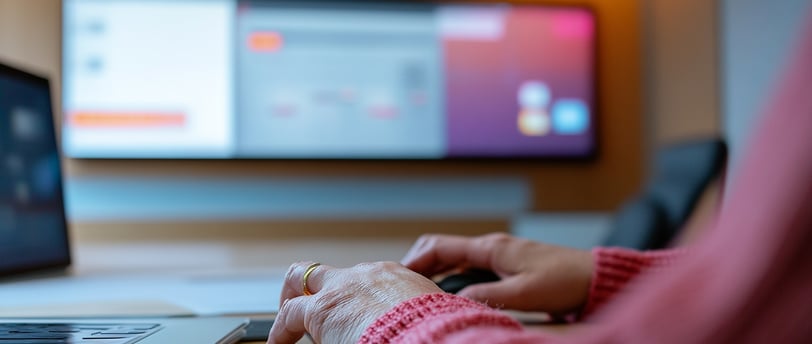
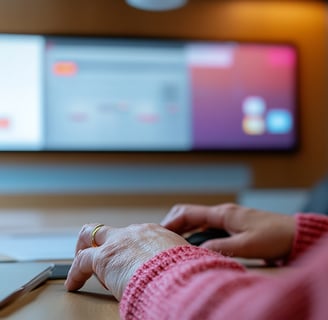
Imagine a world where artificial intelligence can provide accurate, up-to-date information by seamlessly integrating external data sources. This is not a distant dream but a reality made possible by Retrieval-Augmented Generation (RAG). RAG is revolutionizing the way generative AI models operate, enhancing their accuracy and reliability by incorporating real-time data retrieval. In this article, we will explore the concept of RAG, its benefits, and how it is being implemented across various industries.
Understanding Retrieval-Augmented Generation (RAG)
What is RAG?
Retrieval-Augmented Generation (RAG) is an advanced AI framework that combines the strengths of traditional information retrieval systems with the capabilities of generative large language models (LLMs). By integrating external data sources, RAG enables LLMs to provide more accurate, relevant, and up-to-date responses. This process involves retrieving relevant information from external knowledge bases and augmenting the LLM's responses with this data.
How RAG Works
The RAG process can be broken down into several key stages:
Data Preparation and Indexing: All data must be prepared and indexed for use by the LLM. This involves converting data into numerical representations and storing it in a vector database.
Retrieval Phase: When a user inputs a query, the RAG model retrieves the most relevant documents from the vector database. These documents are used to augment the query.
Augmentation Phase: The retrieved data is integrated into the LLM's prompt, enhancing its context and enabling it to generate more accurate responses.
Generation Phase: The LLM generates a response based on both the user query and the retrieved documents. This response can include citations or references to the sources, enhancing user trust1.
Benefits of RAG
RAG offers several advantages over traditional generative AI models:
Improved Accuracy: By retrieving relevant information from external sources, RAG reduces the likelihood of generating inaccurate or outdated responses.
Enhanced User Trust: RAG enables LLMs to provide responses with citations or references, allowing users to verify the information.
Cost-Effective Implementation: RAG eliminates the need for retraining LLMs with additional datasets, making it a more cost-effective solution23.
Applications of RAG
Healthcare
In the healthcare industry, RAG can be used to create AI-powered assistants that provide accurate medical information. For example, a generative AI model supplemented with a medical index could assist doctors and nurses by retrieving relevant medical literature, guidelines, and patient records. This ensures that healthcare professionals have access to the most up-to-date information, improving patient care and outcomes4.
Finance
Financial analysts can benefit from RAG by using AI assistants linked to market data. These assistants can retrieve real-time financial information, news, and market trends, providing analysts with the insights they need to make informed decisions. This application of RAG can lead to more accurate financial forecasts and better investment strategies4.
Customer Support
Businesses can use RAG to enhance their customer support services. By integrating RAG with customer service chatbots, businesses can provide more accurate and relevant responses to customer queries. For example, a customer support chatbot can retrieve information from a company's knowledge base, including FAQs, product manuals, and customer service guides, to provide comprehensive and up-to-date answers to customer questions5.
Implementing RAG
Getting Started with RAG
To implement RAG, businesses can use various tools and platforms that support the framework. For example, NVIDIA provides a reference architecture for building retrieval-augmented generation pipelines. This architecture includes tools like NVIDIA NeMo Retriever, which offers large-scale retrieval accuracy, and NVIDIA NIM microservices for secure, high-performance AI deployment. Additionally, NVIDIA offers a free, hands-on LaunchPad lab for building AI chatbots with RAG, enabling fast and accurate responses from enterprise data4.
Challenges and Solutions
While RAG offers numerous benefits, it also presents challenges. One of the main challenges is ensuring that the retrieved information is accurate and up-to-date. To address this, businesses can implement automated real-time processes or periodic batch processing to update the vector databases with the latest information. Additionally, businesses can use semantic search technologies to enhance the relevance of the retrieved information and improve the quality of the RAG payload5.
Conclusion
Retrieval-Augmented Generation (RAG) is revolutionizing the way generative AI models operate by enhancing their accuracy and reliability through real-time data retrieval. With applications across various industries, including healthcare, finance, and customer support, RAG is becoming an essential tool for businesses looking to leverage the power of generative AI. As the technology continues to evolve, we can expect to see even more innovative uses of RAG, further enhancing the capabilities of AI-powered solutions.
Embrace the future of generative AI with RAG and unlock the potential of accurate, up-to-date, and trustworthy AI responses.
FAQ Section
Q: What is Retrieval-Augmented Generation (RAG)? A: Retrieval-Augmented Generation (RAG) is an AI framework that combines traditional information retrieval systems with generative large language models (LLMs) to provide more accurate and up-to-date responses.
Q: How does RAG work? A: RAG works by retrieving relevant information from external knowledge bases and augmenting the LLM's responses with this data. The process involves data preparation, retrieval, augmentation, and generation phases.
Q: What are the benefits of using RAG? A: RAG offers improved accuracy, enhanced user trust, cost-effective implementation, and the ability to provide up-to-date information. It also eliminates the need for retraining LLMs with additional datasets.
Q: What industries can benefit from RAG? A: Industries such as healthcare, finance, and customer support can benefit from RAG. It can be used to create AI-powered assistants that provide accurate and up-to-date information.
Q: How can businesses implement RAG? A: Businesses can implement RAG using tools and platforms that support the framework, such as NVIDIA's reference architecture for building retrieval-augmented generation pipelines.
Q: What are the challenges of implementing RAG? A: One of the main challenges is ensuring that the retrieved information is accurate and up-to-date. Businesses can address this by implementing automated real-time processes or periodic batch processing to update the vector databases.
Q: How does RAG enhance user trust? A: RAG enhances user trust by enabling LLMs to provide responses with citations or references, allowing users to verify the information.
Q: Can RAG be used to improve customer support services? A: Yes, RAG can be used to enhance customer support services by integrating it with customer service chatbots. This allows businesses to provide more accurate and relevant responses to customer queries.
Q: How does RAG improve the accuracy of AI responses? A: RAG improves the accuracy of AI responses by retrieving relevant information from external sources and augmenting the LLM's responses with this data. This reduces the likelihood of generating inaccurate or outdated responses.
Q: Is RAG a cost-effective solution for businesses? A: Yes, RAG is a cost-effective solution as it eliminates the need for retraining LLMs with additional datasets, making it a more affordable option for businesses.
Additional Resources
Google Cloud - What is Retrieval-Augmented Generation (RAG)?
IBM Research - What is retrieval-augmented generation (RAG)?
Author Bio
Emma Thompson is a technology enthusiast and writer with a background in artificial intelligence and data science. She has a passion for exploring the latest advancements in AI and sharing her insights with others.