What is the role of AI in Inventory Optimization?
The integration of AI technology in Inventory Optimization is a game-changer! Efficient inventory management is essential for any business looking to satisfy customers, cut costs, and increase profits. With AI, you can take your inventory management to the next level and achieve unprecedented success.
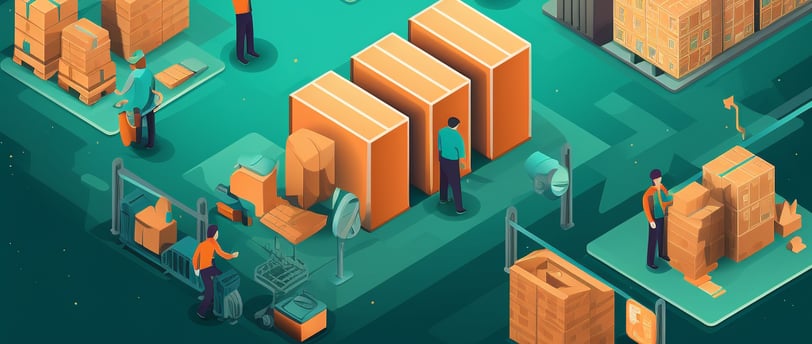
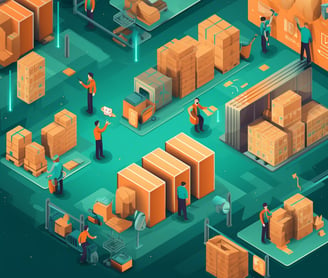
Inventory management is an essential aspect of any business, with the goal of maintaining customer satisfaction while minimizing costs and maximizing profitability. Traditional inventory optimization methods have relied on manual forecasting and decision-making processes, which can be time-consuming, prone to errors, and inefficient. However, artificial intelligence technologies offer a revolutionary approach to improving efficiency and effectiveness in inventory management.[1][2][3]
In this article, we will explore how AI can enhance inventory optimization by addressing its key challenges and delivering significant benefits for businesses. We will also discuss important insights that are crucial to achieving success in this area. Additionally, we will introduce Datasumi's advanced AI-powered platform for inventory management as a valuable tool that enables businesses to leverage the capabilities of AI effectively.
Understanding Inventory Optimization
Inventory optimization is the process of achieving a balance between meeting customer demand and minimizing costs associated with excess inventory. It involves analyzing historical sales data, demand patterns, lead times, and other relevant factors to make informed decisions about replenishment, stock levels, and order quantities. In the past, inventory optimization relied on statistical forecasting models based on historical data and assumptions about future demand. However, these methods often fell short in accounting for the complexities and uncertainties of real-world business environments. This is where AI can be valuable by providing advanced capabilities that enhance inventory optimization beyond traditional approaches.
Achieving optimal inventory control involves striking a delicate balance between satisfying customer demands while concurrently minimizing unnecessary expenses incurred from excessive stock-holding overheads. The analysis includes an examination of previous sales records as well as studying trends relating to market fluctuations over designated periods coupled with utilizing information concerning lead times required for procuring supplies or materials; which subsequently informs better decision-making pertaining to getting products back on shelves rapidly without having surplus amounts unsold at any one given time.[4][5][6]
Historically this practice would have solely been regarded through scrutinizing numerically led predictors used explicitly in understanding purchasing thresholds moving forward; but modern day obstacles pose significant hurdles surpassing mere reliance upon prior success indicators alongside predicting forthcoming consumer habits accurately fully taking into account the complexities and uncertainties of the business landscape.[7]
What is AI & Machine Learning?
When it comes to inventory optimization, AI and machine learning play a crucial role in revolutionizing the way businesses manage their stock levels and meet customer demand. By leveraging these technologies, companies can gain valuable insights into consumer behavior, market trends, and external factors that influence demand for their products.[8][9]
One key area where AI excels in inventory optimization is demand forecasting. Traditional methods rely heavily on historical sales data and statistical models, which may not capture all relevant patterns and fluctuations in demand. With AI-powered machine learning algorithms, businesses can analyze vast amounts of data from various sources and detect hidden patterns and correlations that humans might overlook. This allows for more accurate and dynamic demand forecasts, enabling companies to better align their inventory levels with expected customer needs.[10][11]
Moreover, AI can also improve the efficiency of demand planning by automating processes that were previously manual and time-consuming. For instance, AI algorithms can quickly analyze numerous variables such as past sales performance, promotional activities, seasonality, and economic indicators to generate accurate demand plans. This eliminates the need for labor-intensive calculations and reduces the risk of human error.[12]
Another benefit of AI in inventory optimization is its ability to consider multiple factors simultaneously. Unlike traditional approaches that rely on predetermined formulas or assumptions about future demand, AI algorithms can take into account a wide range of internal and external factors that impact inventory management. These include supplier lead times, production capacity, transportation costs, market trends, competitor analysis, and even weather conditions. By considering all these variables together, AI can provide a more holistic view of inventory needs and enable companies to optimize their stock levels accordingly.[13]
Additionally, AI can support businesses in making strategic decisions regarding their inventory. For example, it can help identify slow-moving or obsolete items that are tying up valuable warehouse space and capital. By analyzing sales data, market trends, and other relevant factors, AI algorithms can provide recommendations on when to phase out certain products or adjust pricing strategies to move inventory more effectively.[14]
AI and machine learning have a significant role to play in inventory optimization. They offer advanced capabilities that surpass traditional methods by leveraging vast amounts of data, detecting hidden patterns, automating processes, considering multiple factors simultaneously, and providing actionable insights for strategic decision-making. As businesses continue to embrace the power of AI, they can expect more streamlined and efficient inventory operations, reduced costs, improved customer satisfaction, and ultimately, a competitive advantage in today's dynamic marketplace.[15][11][16]
How to make Demand Forecasting with AI?
To make demand forecasting with AI, you need to follow several steps. Firstly, gather high-quality data from various sources including sales records, customer feedback, marketing campaigns, and industry reports. This data should cover a long enough period to capture different seasons, trends, and fluctuations. Next, clean and preprocess the data to remove any outliers or inconsistencies that could affect the accuracy of the forecasts.
Once the data is ready, you can start applying machine learning models and algorithms to train your forecasting model. These models use complex mathematical formulas and patterns in the data to identify correlations, dependencies, and trends. By analyzing past demand patterns and their underlying causes, AI can uncover hidden insights that may not be obvious to human analysts.[17][18]
After training the model, you can integrate it into your inventory management system or software. This allows you to continuously update and improve your forecasts based on real-time data inputs. The AI-powered forecasting solution will provide you with more accurate predictions for each product SKU, enabling you to plan procurement, production, and distribution activities more effectively.
One example of how AI can enhance demand forecasting is by identifying seasonality patterns in consumer buying behavior. For instance, if you sell swimwear, AI can recognize that demand typically increases during summer months and decreases during winter. With this insight, you can adjust your inventory levels accordingly, ensuring that you have enough stock available when customers are most likely to purchase.[19][2]
Another benefit of AI in demand forecasting is its ability to handle large volumes of data effortlessly. Traditional approaches often struggle with big datasets and require manual effort to process and analyze them. In contrast, AI algorithms can handle vast amounts of information quickly and efficiently, making it easier to identify patterns and make accurate predictions.
Moreover, AI can consider various influencing factors that may impact demand, such as price changes, competitor activity, or product quality. By incorporating these external variables into the forecasting model, you can get a more holistic understanding of customer demand and adjust your inventory strategy accordingly.[20]
AI plays a crucial role in inventory optimization by enhancing demand forecasting. It enables businesses to utilize machine learning algorithms and advanced analytics techniques to predict future demand patterns with higher accuracy. By considering various factors and handling large volumes of data effortlessly, AI helps optimize stock levels, minimize excess inventory carrying costs, and improve overall supply chain efficiency.[10][21]
Scaling Demand Forecasting with AI
One of the best practices in scaling demand forecasting with AI is to leverage different data sources. Instead of relying solely on past sales data, businesses can consider incorporating additional sources such as market trends, customer feedback, social media analytics, and economic indicators. By considering a wider range of factors, AI algorithms can create more robust and comprehensive demand plans.[22]
Another important aspect is to continuously update and adapt the demand planning models. Demand patterns can change due to various factors like seasonality, new product launches, promotions, or unexpected events. To ensure reliable forecasts, AI algorithms need to be regularly trained and updated with the most current data. This allows them to capture any changes in customer preferences or market dynamics, enabling businesses to make better-informed decisions.[23][24]
Furthermore, collaboration within cross-functional teams is essential for successful implementation. It is important for business stakeholders, supply chain professionals, and data scientists to work together closely to build a scalable and efficient AI-driven demand planning solution. By combining their expertise and insights, they can identify the most valuable components and ingredients for an effective solution.
Moreover, AI-powered tools streamline the demand planning process by automating repetitive tasks, reducing manual effort, and providing real-time reports and insights. These tools enable businesses to focus on strategic decision-making rather than getting caught up in routine tasks. With AI-generated demand forecasts, businesses can set realistic inventory levels, optimize order quantities, and minimize stockouts or excess inventory situations.[21][25]
The role of AI in inventory optimization is significant. Through advanced machine learning algorithms and data analysis techniques, AI enhances demand forecasting capabilities and overcomes the limitations of traditional methods. By scaling demand forecasting with AI, businesses can achieve more accurate predictions, incorporate diverse data sources, continuously adapt to changing demand patterns, and streamline the planning process. Ultimately, AI empowers businesses to make informed decisions about inventory management, ensuring they have enough stock to meet customer demand while minimizing carrying costs.[26][27]
What is Inventory Optimization?
Before delving into the role of AI in inventory optimization, it is crucial to understand the key concerns and challenges that businesses face in this area. Some of the common problems include:
1. Demand Variability: Fluctuations in customer demand can pose a significant challenge for inventory management. Businesses need to forecast demand to avoid stockouts or excessive inventory levels accurately.
2. Seasonality and Trends: Seasonal demand patterns and evolving trends require businesses to adapt their inventory strategies accordingly. Failing to do so can result in missed sales opportunities or excess stock during off-peak periods.
3. Lead Time Variability: Unpredictable lead times from suppliers can impact inventory levels and customer satisfaction. Managing lead time variability effectively is essential for optimizing stock levels and minimizing stockouts.
4. Cost Considerations: Inventory carrying costs, including storage, insurance, and obsolescence, can significantly impact a company's profitability. Balancing cost considerations with customer service levels is a crucial aspect of inventory optimization.
5. SKU Proliferation: As businesses expand their product offerings, managing many stock-keeping units (SKUs) becomes increasingly complex. It requires efficient processes to determine optimal inventory levels for each SKU. [28]
The Role of AI in Inventory Optimization
AI technologies, including machine learning and predictive analytics, are revolutionizing inventory optimization by providing businesses with sophisticated tools to tackle the abovementioned challenges. Here are some key ways in which AI enhances inventory optimization:
1. Accurate Demand Forecasting: AI algorithms can analyze large volumes of historical sales data, incorporating factors such as seasonality, trends, and external events, to generate accurate demand forecasts. Machine learning models can continuously learn from new data, improving forecast accuracy.
2. Dynamic Replenishment Strategies: AI can adjust replenishment strategies based on real-time demand signals and lead time variations. Businesses can optimize order quantities and reorder points by leveraging machine learning algorithms to minimize stockouts and excess inventory.
3. Seasonality and Trend Analysis: AI algorithms can identify seasonal demand patterns and evolving trends by analyzing historical data. This enables businesses to adjust their inventory strategies proactively, ensuring optimal stock levels during peak and off-peak periods.
4. Supplier Performance Monitoring: AI-powered inventory systems can track supplier performance, including lead times and order fulfillment rates. By monitoring supplier data, businesses can identify bottlenecks and make informed decisions regarding supplier selection and relationship management.
5. SKU-Level Optimization: AI can analyze historical sales data for individual SKUs and provide insights into optimal inventory levels for each product. This helps businesses manage SKU proliferation efficiently, avoiding stockouts and excess inventory for specific items.[29][30]
What is the Top-Down / Bottom-up / Middle-out approach?
On the other hand, the Bottom-Up approach focuses on analyzing individual products or SKUs (stock keeping units) to determine their demand patterns. It enables businesses to understand the demand for each specific item and adjust their inventory levels accordingly. The Bottom-Up approach is particularly useful when dealing with unique or niche products that may have different demand patterns compared to the rest of the market.
Lastly, the Middle-Out approach combines elements of both the Top-Down and Bottom-Up approaches. It aims to strike a balance between the macro view and the micro view of inventory optimization. This method considers both the overall market demand as well as the specific demand patterns for individual products or categories. It allows businesses to take advantage of economies of scale while also addressing the specific needs and preferences of customers. The Middle-Out approach offers a comprehensive perspective on inventory optimization, incorporating both strategic decision-making and detailed analysis.
The role of AI in inventory optimization is crucial for businesses seeking to improve their efficiency and profitability. By utilizing AI-powered tools and strategies, businesses can make informed decisions about inventory management, ensuring they meet customer demand while minimizing carrying costs.[31]
Potential Benefits for Businesses
A benefit of AI in inventory optimization is to streamline operations. AI-powered tools can automate various tasks involved in inventory management, such as order placement, replenishment calculations, and stock allocation. This eliminates the need for manual intervention and reduces human error, making the process more efficient and accurate. Businesses can save time and effort by relying on AI algorithms to handle routine inventory tasks, allowing employees to focus on higher-value activities that require critical thinking and problem-solving skills.
Furthermore, AI can help businesses adapt to unexpected events or disruptions. For example, during the COVID-19 pandemic, many businesses experienced sudden changes in customer demand and supply chain disruptions. AI-based demand planning models can quickly adjust forecasts based on real-time data and provide insights into how to respond effectively. By leveraging AI, businesses can make agile decisions to optimize their inventory levels and minimize losses caused by unforeseen circumstances.[32][33]
AI plays a crucial role in inventory optimization by providing businesses with powerful tools and strategies to improve efficiency and profitability. From accurate demand forecasting to streamlining operations and adapting to changing circumstances, AI empowers businesses to make informed decisions that align with customer demand while minimizing costs. By embracing AI-driven solutions, businesses can gain a competitive edge in today's dynamic marketplace and meet the ever-evolving expectations of customers. Here are some of the other benefits:
1. Improved Customer Service: Accurate demand forecasting and optimized stock levels ensure businesses can promptly meet customer demand. This leads to improved customer satisfaction and loyalty.
2. Cost Reduction: AI-driven inventory optimization can minimize excess inventory and associated carrying costs. Businesses can significantly improve their bottom line by avoiding stockouts and reducing holding costs.
3. Enhanced Operational Efficiency: Automation and AI algorithms streamline inventory management processes, reducing manual effort and minimizing errors. This frees up resources for more strategic tasks, improving overall operational efficiency.
4. Adaptive Decision-Making: AI algorithms continuously learn from new data, allowing businesses to make adaptive and data-driven decisions. This ensures that inventory strategies remain aligned with evolving market dynamics.
5. Competitive Advantage: Businesses that leverage AI for inventory optimization gain a competitive edge by improving customer service, reducing costs, and enhancing operational efficiency. This enables them to outperform competitors in an increasingly competitive marketplace.
How Datasumi Can Help
Datasumi, delivers AI-powered inventory management services, offers businesses a comprehensive suite of tools and capabilities to optimize their inventory operations. Leveraging advanced AI algorithms, Datasumi provides the following features: [32]
1. Demand Forecasting: Datasumi uses machine learning models to generate accurate demand forecasts, incorporating historical data, seasonality, and trends. This enables businesses to make data-driven decisions and optimize their inventory levels.
2. Real-Time Inventory Tracking: Datasumi provides real-time visibility into inventory levels, allowing businesses to monitor stock levels across multiple locations and channels. This helps in identifying potential stockouts or excess inventory situations.
3. Automated Replenishment: Datasumi's AI algorithms automatically adjust replenishment strategies based on real-time demand signals and lead time variations. This ensures that businesses can maintain optimal stock levels and minimize stockouts.
4. Supplier Performance Monitoring: Datasumi tracks supplier performance metrics, including lead times and order fulfillment rates, to help businesses identify and manage supply chain bottlenecks effectively.
5. SKU-Level Optimization: Datasumi's AI-powered system analyzes historical sales data for individual SKUs and provides actionable insights for optimizing inventory levels. This enables businesses to manage SKU proliferation efficiently.
Conclusion
AI revolutionizes inventory optimization, offering businesses cutting-edge capabilities to enhance forecasting accuracy, tailor replenishment strategies, and optimize inventory levels. By harnessing the power of AI technologies, businesses can elevate customer service standards, trim costs efficiently, bolster operational efficiency distinguishment while establishing a competitive edge in their respective industries.
Choosing Datasumi as your partner will equip your business with an extensive suite of AI-driven services specifically designed for efficient inventory management. Leveraging Datasumi's exceptional capabilities empowers businesses to tap into the potential of AI and drive substantial improvements in optimizing inventories - ultimately translating into amplified profitability and resounding success within today's dynamic and ever-evolving business sphere.
References
[1] Why Effective And Efficient Inventory Management Is Key To ... - Forbes. [Online]. Available: https://www.forbes.com/sites/forbestechcouncil/2022/04/19/why-effective-and-efficient-inventory-management-is-key-to-delivering-positive-customer-experiences-in-retail/
[2] What is inventory management and how does it work? | IBM. [Online]. Available: https://www.ibm.com/topics/inventory-management
[3] How To Manage Inventory Effectively (2023 Guide) - Forbes. [Online]. Available: https://www.forbes.com/advisor/business/software/how-to-manage-inventory/
[4] Supply Chain Strategy: 4 ways to improve yours - QuickBooks. [Online]. Available: https://quickbooks.intuit.com/global/resources/inventory/supply-chain-strategy/
[5] Ten ways to improve inventory management - Bain & Company. [Online]. Available: https://www.bain.com/insights/ten-ways-to-improve-your-inventory-management-wsj/
[6] What Is Demand Management: Functions, Process and Examples. [Online]. Available: https://www.netsuite.com/portal/resource/articles/inventory-management/demand-management.shtml
[7] Scrutinize Definition & Meaning - Merriam-Webster. [Online]. Available: https://www.merriam-webster.com/dictionary/scrutinize
[8] Succeeding in the AI supply-chain revolution | McKinsey. [Online]. Available: https://www.mckinsey.com/industries/metals-and-mining/our-insights/succeeding-in-the-ai-supply-chain-revolution
[9] Demand forecasting in supply chain technology | McKinsey. [Online]. Available: https://www.mckinsey.com/capabilities/operations/our-insights/to-improve-your-supply-chain-modernize-your-supply-chain-it
[10] 5 Ways AI Can Benefit Demand Forecasting And Inventory Planning - Forbes. [Online]. Available: https://www.forbes.com/sites/forbestechcouncil/2023/02/06/5-ways-ai-can-benefit-demand-forecasting-and-inventory-planning/
[11] AI in Inventory Management: Putting AI to work to optimize inventory. [Online]. Available: https://hypersonix.ai/blog/ai-in-inventory-management/
[12] How AI Is Helping Companies Redesign Processes - Harvard Business Review. [Online]. Available: https://hbr.org/2023/03/how-ai-is-helping-companies-redesign-processes
[13] Inventory Management and Artificial Intelligence | IMPO. [Online]. Available: https://www.impomag.com/inventory-management/article/13249224/inventory-management-and-artificial-intelligence
[14] Using Artificial Intelligence To Improve Business Decisions - Forbes. [Online]. Available: https://www.forbes.com/sites/forbesfinancecouncil/2022/06/21/using-artificial-intelligence-to-improve-business-decisions/
[15] Artificial intelligence, machine learning and deep learning in advanced ... [Online]. Available: https://www.sciencedirect.com/science/article/pii/S2667241323000113
[16] Managing AI Decision-Making Tools - Harvard Business Review. [Online]. Available: https://hbr.org/2021/11/managing-ai-decision-making-tools
[17] AI-driven operations forecasting in data-light environments. [Online]. Available: https://www.mckinsey.com/capabilities/operations/our-insights/ai-driven-operations-forecasting-in-data-light-environments
[18] How To Improve Demand Forecasting With Machine Learning And Real-Time Data. [Online]. Available: https://www.forbes.com/sites/forbestechcouncil/2022/04/26/how-to-improve-demand-forecasting-with-machine-learning-and-real-time-data/
[19] Inventory Management System Basics | Microsoft Dynamics 365. [Online]. Available: https://dynamics.microsoft.com/en-us/field-service/inventory-management-system/
[20] 7 factors that influence the demand of consumer goods - NIQ. [Online]. Available: https://nielseniq.com/global/en/insights/analysis/2022/7-factors-that-influence-the-demand-of-consumer-goods/
[21] Better supply-chain planning with AI and machine learning | McKinsey. [Online]. Available: https://www.mckinsey.com/capabilities/operations/our-insights/autonomous-supply-chain-planning-for-consumer-goods-companies
[22] AI Can Help Companies Tap New Sources of Data for Analytics. [Online]. Available: https://hbr.org/2021/03/ai-can-help-companies-tap-new-sources-of-data-for-analytics
[23] Demand Planning: What It Is and Why It’s Important | NetSuite. [Online]. Available: https://www.netsuite.com/portal/resource/articles/erp/demand-planning.shtml
[24] A Simpler Way to Modernize Your Supply Chain - Harvard Business Review. [Online]. Available: https://hbr.org/2021/09/a-simpler-way-to-modernize-your-supply-chain
[25] AI, automation, and the future of work: Ten things to solve for. [Online]. Available: https://www.mckinsey.com/featured-insights/future-of-work/ai-automation-and-the-future-of-work-ten-things-to-solve-for
[26] Three Inventory Challenges Solved with AI and Machine Learning. [Online]. Available: https://blogs.oracle.com/retail/post/three-inventory-challenges-solved-with-ai-and-machine-learning
[27] Inventory Optimization Using Machine Learning Algorithms. [Online]. Available: https://link.springer.com/chapter/10.1007/978-981-16-6460-1_41
[28] Explore the impact of demand… | bartleby. [Online]. Available: https://www.bartleby.com/questions-and-answers/explore-the-impact-of-demand-variability-on-the-effectiveness-of-the-wilson-approach/3947ecd6-20db-4cd3-b8d8-7a1bfa5c9792
[29] Demand Forecasting Software: Demystifying AI. [Online]. Available: https://www.logility.com/blog/demand-forecasting-software-demystifying-ai/
[30] Demand forecasting: AI-based, statistical and hybrid models vs ... [Online]. Available: https://www.researchgate.net/publication/367090536_DEMAND_FORECASTING_AI-BASED_STATISTICAL_AND_HYBRID_MODELS_VS_PRACTICE-BASED_MODELS-THE_CASE_OF_SMES_AND_LARGE_ENTERPRISES
[31] Explain about top-down approach and bottom-up approach with... [Online]. Available: https://www.coursehero.com/tutors-problems/Object-Oriented-Programming/52692596-Explain-about-top-down-approach-and-bottom-up-approach-with/
[32] Unlocking The Power Of Predictive Analytics With AI - Forbes. [Online]. Available: https://www.forbes.com/sites/forbestechcouncil/2021/08/11/unlocking-the-power-of-predictive-analytics-with-ai/
[33] AI for supply chain can help companies handle disruptions. [Online]. Available: https://www.techtarget.com/searchenterpriseai/feature/AI-for-supply-chain-can-help-companies-handle-disruptions