Amazon SageMaker: Your All-in-One Platform for Machine Learning
Discover the game-changing power of Machine Learning (ML) with Amazon SageMaker! 🚀💡 Unlock invaluable insights from your data 📊 and make informed decisions that will propel your business 📈 to new heights. SageMaker is your one-stop-shop 🛒 for all your ML needs! 🤖
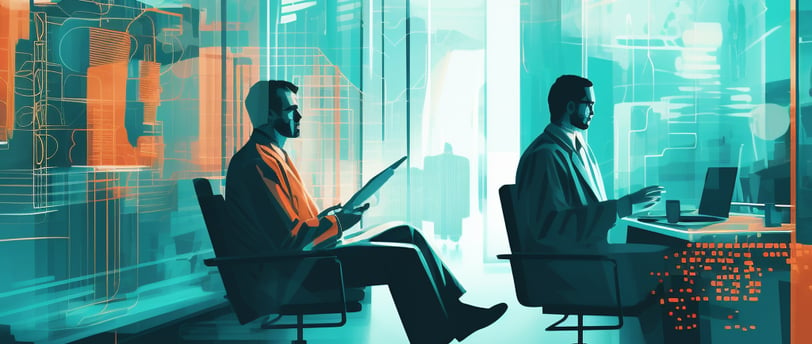
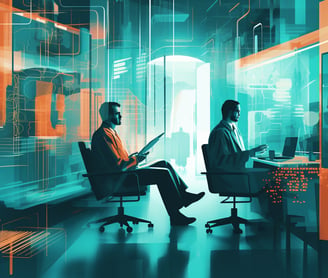
Machine Learning (ML) has emerged as a powerful tool for businesses to gain insights from their data and make informed decisions. However, implementing ML models can be complex and challenging, requiring specialized skills, infrastructure, and resources. To simplify this process, Amazon Web Services (AWS) developed Amazon SageMaker, an all-in-one platform for machine learning. In this article, we will delve into the depths of Amazon SageMaker, exploring its key features, benefits for businesses, and how Datasumi can assist in leveraging this platform for success.
Understanding Amazon SageMaker
Amazon SageMaker is a fully managed service that provides tools and capabilities to build, train, deploy, and manage ML models. It aims to remove the complexities associated with ML development, making it accessible to a broader audience. The platform integrates seamlessly with other AWS services, offering a unified environment for data scientists, developers, and business stakeholders.[1][2][3][4][5]
Comprehensive Overview of Amazon SageMaker's Key Features
Amazon SageMaker is a fully managed machine learning service that empowers data scientists, developers, and machine learning practitioners to build, train, and deploy machine learning models at scale. Designed to remove the heavy lifting from the machine learning process, SageMaker offers an array of robust features to make model development and deployment easier, faster, and more efficient. Let's delve into the platform's standout features:[6][7][4][8][1]
Notebook Instances for Streamlined Development
SageMaker boasts Jupyter Notebook instances designed to facilitate the collaborative development of machine learning models. These instances are pre-configured with all essential libraries and frameworks. This relieves users of the hassles of infrastructure setup, enabling them to focus solely on creating effective and efficient models.[9][10][11][12]
Suite of Built-in Algorithms
SageMaker offers a comprehensive set of built-in algorithms that are optimized for various machine learning tasks like regression, classification, and clustering. These algorithms are designed for high performance and scalability, eliminating the need for developers to build algorithms from scratch. This reduces both the time and resources needed for training effective models.[1][13]
Flexibility through Custom Algorithm Support
The platform doesn't limit you to its built-in algorithms; SageMaker allows for the integration of custom algorithms and frameworks. This offers data scientists the flexibility to utilize the tools they are most comfortable with while benefiting from SageMaker’s robust, scalable infrastructure.[2][14]
User-friendly Data Labeling
Labeling vast datasets can often be a cumbersome and labour-intensive process. SageMaker alleviates this by offering an intuitive data labeling feature. The platform provides a user-friendly interface for completing annotation tasks, substantially speeding up the data preparation phase, which is essential for training high-accuracy machine learning models.[15][16]
Automatic Hyperparameter Optimization
One of the most vital steps in achieving peak model performance is hyperparameter tuning. SageMaker excels in this area by offering automated model tuning features. The platform uses built-in algorithms for hyperparameter optimization, which spares you the manual labor, saving both time and computational resources and ensuring that your models perform at their best.[17][18]
Scalable and Reliable Model Hosting
After your machine learning model is trained and ready, SageMaker facilitates quick deployment for inference. The platform provides a robust, scalable, and highly available infrastructure to host your machine learning models. This ease of integration makes it straightforward to incorporate these models into production-level applications.
Amazon SageMaker is a powerful, flexible, and user-friendly platform for machine learning development. Its features are carefully designed to facilitate each step of the machine learning pipeline, from development and training to deployment, ensuring that you get the most out of your machine learning projects.
Unpacking the Solutions Offered by Amazon SageMaker: Addressing Key Pain Points in Machine Learning Operations
Amazon SageMaker emerges as a one-stop solution for businesses looking to overcome the typical challenges associated with machine learning (ML) development and deployment. As ML projects transition from experiments to critical business components, companies face myriad issues ranging from infrastructure management to skill gaps. SageMaker offers a comprehensive set of features designed to alleviate these concerns, thus accelerating your ML journey and driving innovation. Below we delve into some of the key areas where SageMaker stands out:[19]
Simplified Infrastructure Management
One of the most overwhelming aspects of ML development is the initial setup and ongoing management of the necessary infrastructure. Amazon SageMaker drastically simplifies this process by taking on the heavy lifting of provisioning compute resources, ensuring scalability, and overseeing maintenance tasks. This service offering means that businesses can channel their energies into the core aspects of model development and data experimentation rather than getting bogged down with infrastructure concerns.[20][21]
Bridging the Skill Gap
ML projects often require a unique set of specialized skills and technical expertise, which can be a barrier to entry for many businesses. SageMaker democratises access to machine learning by offering a user-friendly interface that streamlines the development process. This inclusivity enables data scientists, developers, and even business stakeholders with limited ML knowledge to harness the platform's capabilities effectively. Essentially, SageMaker reduces the steep learning curve often associated with ML development.[22][23]
Ensuring Scalability for High-Volume Data
Training ML models on voluminous datasets can be a daunting task that consumes both time and computational resources. SageMaker addresses this issue head-on by offering a robust, scalable infrastructure that can handle large-scale training tasks efficiently. Integrated with the powerful elastic computing capabilities of AWS, SageMaker enables organizations to train sophisticated models faster, allowing for quicker iterations and more efficient use of resources.[24][25][26][27]
Cost-Effective Operations
Setting up a traditional machine learning infrastructure can be not only time-consuming but also financially burdensome. SageMaker revolutionizes this by offering a flexible, pay-as-you-go pricing model. Users are only billed for the computing power and storage space they actually use, making it an ideal solution for businesses looking to keep costs in check. This flexibility empowers organizations to scale their resources up or down based on specific project requirements, thus optimizing operational costs.[28][29]
Amazon SageMaker addresses the most pressing concerns of ML operations, offering businesses a streamlined, cost-effective, and scalable solution. By mitigating challenges in infrastructure management, skill requirements, data scalability, and cost efficiency, SageMaker enables organizations to focus on what truly matters: deriving valuable insights and innovation from their data.
Unleashing Business Potential with Amazon SageMaker
In the data-driven business landscape, deploying robust machine learning (ML) solutions is more critical than ever for achieving a competitive edge. Amazon SageMaker serves as a versatile platform that simplifies the complexities involved in ML model development, from concept to deployment. By providing an integrated environment, SageMaker accelerates the journey of turning raw data into actionable insights. Below are some of the game-changing benefits businesses can expect when they integrate Amazon SageMaker into their operations.[30][31][32]
Speeding Up Machine Learning Development
One of the primary advantages of utilizing Amazon SageMaker is the acceleration of ML development timelines. The platform's cohesive environment comes with pre-packaged algorithms and a streamlined workflow that covers every phase of ML development—ranging from data preprocessing and model training to deployment. This holistic approach substantially reduces the time needed to transition from prototype to production-ready solutions, thereby enabling businesses to respond swiftly to market demands.[33][1][34]
Fostering Team Collaboration for Enhanced Productivity
Amazon SageMaker is engineered to facilitate seamless collaboration between data scientists, engineers, and other stakeholders involved in ML projects. The platform offers collaborative notebook instances where multiple team members can work in real-time on the same project. This capability allows for the sharing of code, research findings, documentation, and visual representations, enhancing the exchange of knowledge and boosting overall team productivity.[35][36][37]
Scalability for Growing Business Needs
Scalability is a significant concern for businesses that are continuously expanding their data and computational needs. Amazon SageMaker addresses this concern by offering a highly scalable infrastructure capable of growing in parallel with business demands. The platform automates the allocation of computing resources based on the size and complexity of the ML workloads. This auto-provisioning ensures that businesses always have the necessary computational power for high performance, while simultaneously achieving efficient resource utilization.[38][39][40]
Achieving Higher Model Accuracy
Model accuracy is paramount for effective ML solutions, and SageMaker has features designed explicitly for this. The platform's capabilities extend to automatic model tuning and hyperparameter optimization. It employs a systematic approach to explore the hyperparameter space, selecting configurations that offer the most promising results. By doing so, Amazon SageMaker significantly improves the performance and accuracy of ML models, making them more reliable for business applications.[14][41][42]
Cost-Efficiency for Budget-Friendly Implementation
Cost often stands as a significant hurdle for businesses aspiring to implement cutting-edge ML solutions. Amazon SageMaker mitigates this challenge by offering a flexible, pay-as-you-go pricing structure. This model enables businesses to incur costs only for the resources they actively use, eliminating the need for substantial upfront investment in hardware and software. As a result, SageMaker removes financial barriers, making it more accessible for businesses to leverage ML and achieve greater return on investment.[25][38][43]
By harnessing the power of Amazon SageMaker, businesses can fast-track their ML initiatives, encourage better team collaboration, scale effortlessly, enhance model accuracy, and maintain budget-friendly operations—all critical components for achieving success in today's competitive marketplace.
Elevate Your Business with Datasumi's Expertise in Data Science and Machine Learning
In today's fast-paced and ever-changing business environment, data-driven decision-making has become increasingly important for success. Datasumi, a leading data science consulting firm, enables companies to fully leverage the power of Machine Learning and Artificial Intelligence. With expertise in Amazon SageMaker and various AWS services, we deliver tailored solutions that offer valuable insights and guidance. Our comprehensive range of services covers everything from strategy development to infrastructure optimization, all aimed at maximizing the effectiveness and return on investment of your SageMaker projects. Discover how Datasumi can help revolutionize your business:
Consulting Services for Strategic Roadmapping
At Datasumi, we provide first-class consulting services to help you crystalize your ML strategy and roadmap. Our team consists of seasoned data scientists and ML engineers who conduct a meticulous assessment of your business needs. Based on this, we recommend the most effective strategies for harnessing the power of SageMaker in your unique operational context.
Bespoke Model Development for Tailored Solutions
Every business is unique, and so should the solutions that drive it. Datasumi offers custom ML model development services that are specifically designed to meet your business requirements. Utilizing the flexibility of SageMaker, our experts can develop and deploy models that not only solve specific challenges in your industry but also offer actionable insights to drive your business forward.
Infrastructure Setup and Resource Optimization
Efficient infrastructure is the backbone of any successful ML initiative. We understand the critical importance of setting up the right infrastructure that aligns with your organizational needs, both in terms of performance and cost-effectiveness. Datasumi's technical team assists in designing and deploying an infrastructure that ensures optimal resource utilization, scalability, and ultimately, a lower total cost of ownership.
Comprehensive Training and Skill Development
Human capital is the most valuable asset in any organization. To ensure that your team is equipped to make the most of SageMaker and other ML tools, Datasumi provides intensive training programs. Our curriculum is structured to empower data scientists, developers, and even business stakeholders with the requisite knowledge and skills. The modules cover everything from data preprocessing and model training to deployment and real-time monitoring.
Sustained Maintenance and Operational Support
An ML model is not a set-it-and-forget-it endeavor. It requires ongoing attention to ensure that it performs optimally and adapts to new data patterns and business goals. Datasumi offers continued maintenance and support services to guarantee the smooth operation of your deployed ML models. This involves close monitoring of model performance, swift troubleshooting of any emerging issues, and timely updates or enhancements to keep your solutions at the cutting edge.
With Datasumi as your partner, you gain a wealth of expertise and a commitment to excellence that will elevate your business to new heights. We help you navigate the complexities of implementing ML and AI solutions, making your journey from strategy to execution seamless and effective.
Conclusion
Amazon SageMaker is a powerful all-in-one platform for machine learning, offering a comprehensive suite of tools and capabilities to simplify the ML development process. By addressing key concerns such as infrastructure management, skill gaps, scalability, and cost optimization, SageMaker empowers businesses to leverage ML's benefits effectively. With the expertise of Datasumi, businesses can unlock the full potential of SageMaker and drive successful ML initiatives. Whether it's consulting services, custom model development, infrastructure optimization, or training and support, Datasumi can assist businesses at every stage of their ML journey, ensuring long-term success and competitive advantage.
References
What is Amazon SageMaker? - Amazon SageMaker. https://docs.aws.amazon.com/sagemaker/latest/dg/whatis.html.
Machine Learning – Amazon SageMaker FAQs – AWS. https://aws.amazon.com/sagemaker/faqs/.
Machine Learning – Amazon Web Services. https://aws.amazon.com/sagemaker/.
AWS Announces Eight New Amazon SageMaker Capabilities - Press Center. https://press.aboutamazon.com/2022/11/aws-announces-eight-new-amazon-sagemaker-capabilities.
Amazon SageMaker Feature Store. https://aws.amazon.com/sagemaker/feature-store/.
AWS Announces Six New Amazon SageMaker Capabilities - Press Center. https://press.aboutamazon.com/2021/12/aws-announces-six-new-amazon-sagemaker-capabilities.
Amazon SageMaker – Accelerating Machine Learning | AWS News Blog. https://aws.amazon.com/blogs/aws/sagemaker/.
How to Build, Train, and Deploy Machine Learning Models with Amazon .... https://aws.amazon.com/machine-learning/accelerate-amazon-sagemaker/.
Step 1: Create an Amazon SageMaker Notebook Instance - docs.aws.amazon.com. https://docs.aws.amazon.com/sagemaker/latest/dg/gs-setup-working-env.html.
Next Generation SageMaker Notebooks - aws.amazon.com. https://aws.amazon.com/blogs/aws/next-generation-sagemaker-notebooks-now-with-built-in-data-preparation-real-time-collaboration-and-notebook-automation/.
Understanding Amazon SageMaker notebook instance networking .... https://aws.amazon.com/blogs/machine-learning/understanding-amazon-sagemaker-notebook-instance-networking-configurations-and-advanced-routing-options/.
Amazon SageMaker Notebook Instances - docs.aws.amazon.com. https://docs.aws.amazon.com/sagemaker/latest/dg/nbi.html.
Introduction to Amazon Machine Learning and SageMaker Algorithms .... https://saturncloud.io/blog/introduction-to-amazon-machine-learning-and-sagemaker-algorithms/.
Use Amazon SageMaker Built-in Algorithms or Pre-trained Models. https://docs.aws.amazon.com/sagemaker/latest/dg/algos.html.
Data Labeling: How AI Can Streamline Your Data Labelling. https://towardsdatascience.com/data-labeling-how-ai-can-streamline-your-data-labelling-4f1ffb8a19e1.
Top 25 Data Labeling Tools Landscape in 2023 | Galliot. https://galliot.us/blog/top-25-data-labeling-tools-landscape-2023/.
Optimize hyperparameters with Amazon SageMaker Automatic Model Tuning .... https://aws.amazon.com/blogs/machine-learning/optimize-hyperparameters-with-amazon-sagemaker-automatic-model-tuning/.
How Hyperparameter Tuning Works - Amazon SageMaker. https://docs.aws.amazon.com/sagemaker/latest/dg/automatic-model-tuning-how-it-works.html.
Accelerate Machine Learning with Amazon SageMaker Ready Partners. https://aws.amazon.com/blogs/apn/accelerate-machine-learning-with-amazon-sagemaker-ready-partners/.
MLOps Principles - ML Ops: Machine Learning Operations. https://ml-ops.org/content/mlops-principles.
Real-time inference - Amazon SageMaker. https://docs.aws.amazon.com/sagemaker/latest/dg/realtime-endpoints.html.
10 Mandatory Skills to Become an AI & ML Engineer - KnowledgeHut. https://www.knowledgehut.com/blog/data-science/skills-of-ai-and-ml-engineer.
21 Machine Learning Projects [Beginner to Advanced Guide] - Springboard. https://www.springboard.com/blog/data-science/machine-learning-projects/.
Tutorial - Train a Machine Learning Model - Amazon Web Services. https://aws.amazon.com/tutorials/machine-learning-tutorial-train-a-model/.
Machine Learning Model Training - Amazon Web Services. https://aws.amazon.com/sagemaker/train/.
Build & train models - Azure Machine Learning | Microsoft Learn. https://learn.microsoft.com/en-us/azure/machine-learning/concept-train-machine-learning-model?view=azureml-api-2.
Handling Large Datasets in Data Preparation & ML Training Using MLOps. https://ai-infrastructure.org/handling-large-datasets-in-data-preparation-ml-training-using-mlops/.
Organize and set up Azure Machine Learning environments. https://learn.microsoft.com/en-us/azure/cloud-adoption-framework/ready/azure-best-practices/ai-machine-learning-resource-organization.
Deep Learning Infrastructure at Scale: An Overview - MLconf. https://mlconf.com/blog/deep-learning-infrastructure-at-scale-an-overview/.
Unlocking the Business Value of Machine Learning—With Organizational .... https://aws.amazon.com/blogs/enterprise-strategy/unlocking-the-business-value-of-machine-learning-with-organizational-learning/.
How The World Became Data-Driven, And What’s Next - Forbes. https://www.forbes.com/sites/googlecloud/2020/05/20/how-the-world-became-data-driven-and-whats-next/.
The 4 Pillars of MLOps: How to Deploy ML Models to Production. https://www.phdata.io/blog/the-ultimate-mlops-guide-how-to-deploy-ml-models-to-production/.
Pros and Cons of Amazon SageMaker 2023 - TrustRadius. https://www.trustradius.com/products/amazon-sagemaker/reviews?qs=pros-and-cons.
Building, automating, managing, and scaling ML workflows using Amazon .... https://aws.amazon.com/blogs/machine-learning/building-automating-managing-and-scaling-ml-workflows-using-amazon-sagemaker-pipelines/.
What is a SageMaker Project? - Amazon SageMaker. https://docs.aws.amazon.com/sagemaker/latest/dg/sagemaker-projects-whatis.html.
Amazon SageMaker Studio now supports real time collaboration. https://aws.amazon.com/about-aws/whats-new/2022/11/amazon-sagemaker-studio-supports-real-time-collaboration/.
Collaborate with data scientists - Amazon SageMaker. https://docs.aws.amazon.com/sagemaker/latest/dg/canvas-collaborate.html.
Machine Learning and Artificial Intelligence - Amazon Web Services. https://aws.amazon.com/machine-learning/.
High Performance Computing (HPC) | AWS. https://aws.amazon.com/hpc/.
Automatically Scale Amazon SageMaker Models. https://docs.aws.amazon.com/sagemaker/latest/dg/endpoint-auto-scaling.html.
Machine Learning (ML) Customers - Amazon Web Services. https://aws.amazon.com/sagemaker/customers/.
Testing approaches for Amazon SageMaker ML models. https://aws.amazon.com/blogs/machine-learning/testing-approaches-for-amazon-sagemaker-ml-models/.
12 Expert Tips To Help Small Businesses Affordably Leverage AI/ML - Forbes. https://www.forbes.com/sites/forbestechcouncil/2021/04/19/12-expert-tips-to-help-small-businesses-affordably-leverage-aiml/.