Can GenAI Flag Potential Fraud in Insurance Claims?
7/21/20247 min read
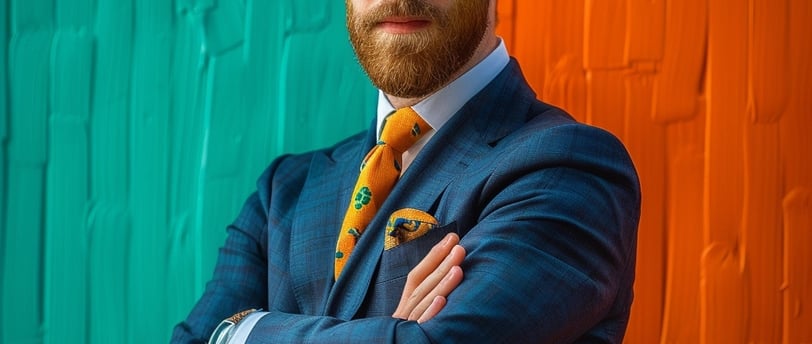
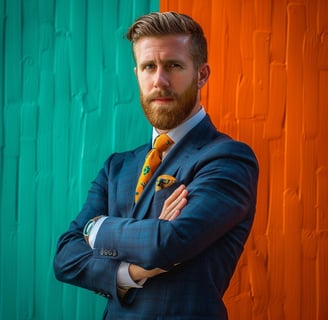
Generative Artificial Intelligence (GenAI) has rapidly emerged as a transformative technology across various industries, offering innovative solutions to complex problems. Its prowess in analyzing vast datasets and recognizing intricate patterns has positioned it as a crucial tool in the realm of fraud detection. In sectors like finance, healthcare, and particularly insurance, where fraudulent activities have become increasingly sophisticated, the need for advanced technological interventions is paramount.
Fraudulent claims in the insurance industry have escalated, costing businesses billions of dollars annually. Traditional methods of fraud detection, which often rely on manual processes and rule-based systems, are proving inadequate in the face of evolving fraudulent tactics. This has driven the insurance sector to seek more robust and intelligent solutions to safeguard their operations. Enter GenAI, with its ability to process and analyze enormous volumes of data, identifying subtle anomalies and patterns that may elude human analysts.
GenAI leverages advanced machine learning algorithms to sift through data, learning from historical claims and identifying characteristics that deviate from the norm. This capability enables it to flag potential fraud with a higher degree of accuracy and speed compared to conventional methods. By continuously learning and adapting to new data, GenAI systems can stay ahead of fraudsters, who are constantly devising new ways to exploit vulnerabilities.
Moreover, GenAI's application in fraud detection is not limited to merely flagging suspicious claims. It also plays a pivotal role in streamlining the investigation process, providing insurance companies with actionable insights and reducing the time and resources required for fraud management. As the insurance industry continues to grapple with the growing threat of fraud, the integration of GenAI presents a promising avenue for enhancing the effectiveness and efficiency of fraud detection mechanisms.
Pattern Recognition Capabilities
Utilizing Generative Artificial Intelligence (GenAI) in the insurance industry significantly enhances the detection of fraudulent activities through its advanced pattern recognition capabilities. By analyzing extensive volumes of claims data, GenAI can identify anomalies that may indicate potential fraud. This process involves a detailed examination of historical claims data to discern common characteristics of fraudulent claims. Consequently, insurers are better equipped to flag suspicious submissions effectively.
The initial step in this process is the aggregation and examination of historical claims data. GenAI systems are designed to sift through vast datasets, identifying trends and discrepancies that human analysts might overlook. These systems learn from past fraud cases, recognizing specific patterns that typically characterize fraudulent activity. For instance, repeated claims for similar incidents or unusually high claim amounts are classic indicators of potential fraud. By understanding these patterns, GenAI can create a robust framework for evaluating new claims.
One practical example is the detection of repeated claims for similar incidents. If an individual or entity submits multiple claims for the same type of loss within a short period, this could be a red flag. GenAI systems are adept at cross-referencing current claims with past data, highlighting such repetitions and allowing investigators to scrutinize further.
Another significant pattern involves unusually high claim amounts. While high claims can sometimes be legitimate, they often warrant closer examination. GenAI can compare the amount claimed against average claim amounts for similar incidents, flagging those that deviate significantly from the norm. This facilitates a more focused review process, ensuring that potentially fraudulent claims are investigated more thoroughly.
Beyond these specific examples, GenAI's pattern recognition capabilities extend to more nuanced indicators, such as the timing and geographical distribution of claims. By continuously learning and adapting to new data, GenAI systems can refine their detection algorithms, improving their accuracy and efficiency over time. This ongoing refinement ensures that insurers remain one step ahead of fraudsters, safeguarding their operations and maintaining the integrity of the claims process.
Inconsistency Detection in Claims
One of the pivotal roles of Generative AI (GenAI) in the insurance sector is its capability to detect inconsistencies in claims. By leveraging advanced algorithms, GenAI can meticulously analyze the information provided by claimants and identify discrepancies with remarkable accuracy. This process involves comparing the details of a claim against a vast array of typical data points, thereby enabling the detection of anomalies that might indicate fraudulent activity.
For instance, GenAI can identify mismatched dates in a claim. Suppose a claimant reports an accident date that does not align with other related events or documented evidence; GenAI can flag this inconsistency for further investigation. Similarly, if the description of an injury in a medical report does not match the narrative provided in the claim, this discrepancy can be detected by the AI system. Moreover, claims that deviate significantly from established normsโsuch as an unusually high number of claims from a particular individual or locationโcan also be highlighted as potential red flags.
The efficiency of GenAI in cross-referencing data from multiple sources is a critical factor in its effectiveness. By integrating information from various databases, including medical records, vehicle logs, and historical claims data, GenAI can build a comprehensive overview of each claim. This holistic approach ensures that any inconsistencies, no matter how subtle, are brought to light. For example, if a claimantโs stated timeline of events conflicts with GPS data or witness statements, GenAI will identify and report this inconsistency.
In essence, the ability of GenAI to detect and analyze inconsistencies in insurance claims not only enhances the accuracy and reliability of fraud detection but also streamlines the claims processing workflow. By automating the identification of potential fraud, GenAI empowers insurance companies to allocate their resources more efficiently and focus on genuine claims, ultimately improving both operational efficiency and customer trust.
Behavioral Analysis and Anomaly Detection
In the realm of insurance claims, behavioral analysis and anomaly detection have emerged as pivotal tools for identifying potential fraud. Generative Artificial Intelligence (GenAI) transcends traditional data comparison methods by delving into the intricate patterns of claimant behavior over an extended period. This sophisticated approach enables the detection of anomalies that may signify fraudulent activity.
GenAI leverages machine learning algorithms to scrutinize behavioral patterns, contrasting them against established norms. By continually analyzing the nuances of claimants' actions, it can pinpoint deviations that might otherwise go unnoticed. For instance, an unusually high frequency of claims or inconsistencies in the claimantโs reported information could trigger alerts for further investigation.
The efficacy of GenAI in behavioral analysis hinges on its ability to learn and adapt. Machine learning algorithms play a critical role here, as they are designed to evolve with the influx of new data. Each new data point refines the algorithms, enhancing their predictive accuracy. Over time, this iterative learning process enables the system to distinguish between genuine claims and those that exhibit suspicious characteristics.
Moreover, GenAI's capability to perform real-time analysis is particularly advantageous. As new claims are filed, the system instantaneously assesses them against a backdrop of historical data, flagging any anomalies for closer scrutiny. This proactive approach not only facilitates the early detection of potential fraud but also contributes to more efficient claim processing workflows.
In essence, the integration of GenAI for behavioral analysis and anomaly detection represents a significant advancement in the fight against insurance fraud. By continuously refining its algorithms and incorporating real-time data, GenAI offers a robust mechanism for safeguarding the integrity of insurance claims processes. Through its meticulous examination of behavioral patterns, it stands as a formidable ally in mitigating fraudulent activities and ensuring the accuracy of claim assessments.
Integration with Human Expertise
The integration of Generative Artificial Intelligence (GenAI) with human expertise is pivotal in enhancing the fraud detection process within insurance claims. While GenAI excels at analyzing vast amounts of data and identifying potential fraud patterns, human adjusters contribute an indispensable layer of contextual understanding and experience. This collaborative approach leverages the strengths of both AI and human judgment to create a more robust and reliable system.
GenAI systems are designed to flag potential fraud cases by sifting through extensive datasets and recognizing anomalies that may indicate fraudulent activity. These systems utilize advanced algorithms and machine learning techniques to detect subtle patterns that might be overlooked by human eyes. However, the role of human adjusters remains crucial in this process. Upon flagging by GenAI, these cases are then reviewed by experienced adjusters who can apply their nuanced understanding of the industry, along with situational and contextual knowledge, to make informed decisions.
The synergy between AI and human expertise ensures a balanced approach to fraud detection. GenAI handles the heavy lifting by processing and analyzing data at a scale and speed unattainable by humans alone. Meanwhile, human adjusters bring in their ability to interpret complex scenarios, consider external factors, and apply ethical considerations that AI systems may not fully grasp. This partnership not only increases the accuracy of fraud detection but also ensures that legitimate claims are processed efficiently, minimizing inconvenience to genuine claimants.
Moreover, the collaborative approach allows for continuous improvement in both AI systems and human decision-making. Feedback from human adjusters can be used to refine and enhance the algorithms, making GenAI even more adept at recognizing fraud patterns over time. Conversely, adjusters can benefit from insights provided by GenAI, gaining a deeper understanding of emerging fraud tactics and trends.
In essence, the integration of GenAI with human expertise represents a balanced and effective strategy in the ongoing battle against insurance fraud. By combining the analytical power of AI with the intuitive and contextual capabilities of humans, the insurance industry can achieve a higher standard of accuracy and efficiency in fraud detection and claims processing.
Challenges and Future of GenAI in Fraud Detection
Implementing Generative AI (GenAI) in fraud detection within the insurance sector presents several challenges. One of the foremost concerns is data privacy. Utilizing extensive datasets for training AI models can potentially compromise sensitive customer information, making robust data anonymization and encryption techniques imperative. Moreover, the need for large datasets poses logistical and operational hurdles, as acquiring and processing such vast amounts of data requires significant resource investment and advanced infrastructure.
Another critical challenge is the risk of generating false positives. An overzealous fraud detection system may flag legitimate claims as fraudulent, leading to customer dissatisfaction and increased operational costs due to unnecessary investigations. Ensuring the precision of GenAI models involves a delicate balance between sensitivity and specificity, requiring ongoing refinement and tuning of algorithms.
Despite these challenges, significant advancements in AI technology are poised to address these issues. Innovations in federated learning, for instance, allow AI models to be trained across decentralized data sources without compromising data privacy. This approach not only protects customer information but also enhances the robustness of the models by incorporating diverse data inputs. Additionally, improvements in natural language processing (NLP) and machine learning algorithms continue to enhance the accuracy and efficiency of fraud detection systems, reducing the likelihood of false positives.
Looking ahead, the future of GenAI in the insurance industry appears promising. As AI technologies evolve, we can expect improvements in the speed and accuracy of fraud detection processes. Enhanced integration capabilities will enable seamless interoperability with other technologies, such as blockchain for secure data sharing and advanced analytics platforms for real-time decision-making. These developments will help insurers better manage fraud risks, streamline operations, and ultimately provide more reliable and efficient services to their customers.
WORK SMARTER WITH DATASUMI
Products & Services
Contact Us โ๏ธ
About us
Terms of Service ๐
GDPR Statement ๐ช๐บ
Learn about
Privacy Policy ๐
Our Blog ๐