How Real-Time Data Analytics Helps in Predicting Demand
7/22/20247 min read
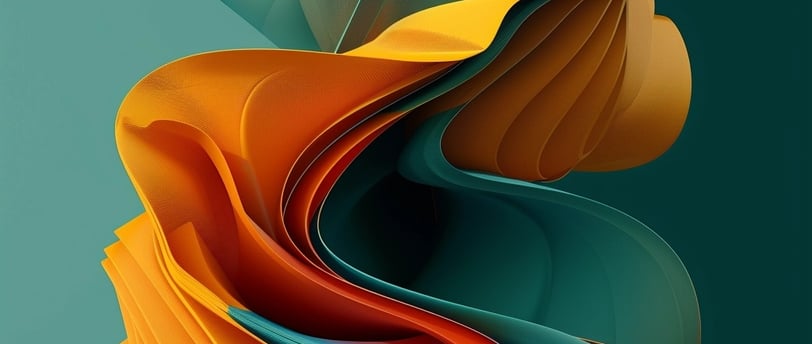
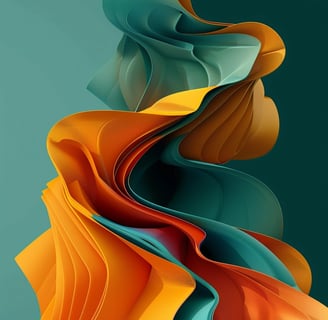
Real-time data analytics play a pivotal role in capturing immediate visibility into current demand patterns. Businesses can identify sudden changes, emerging trends, and seasonal variations as they happen, which is crucial for maintaining competitive advantage. By integrating this timely information into demand forecasting models, companies can significantly enhance the accuracy of their future predictions.
One of the primary sources of real-time data is social media. Platforms like Twitter, Facebook, and Instagram generate a vast amount of user-generated content that can be mined for insights into consumer preferences and emerging trends. For instance, a sudden spike in mentions of a particular product or service can signal a rise in demand, allowing businesses to adjust their inventory and marketing strategies accordingly.
Web traffic is another critical source of real-time data. E-commerce platforms and company websites offer valuable insights into consumer behavior through metrics such as page views, click-through rates, and time spent on specific product pages. Analyzing these metrics helps businesses understand which products are gaining traction and which ones are losing appeal, thus enabling timely adjustments in their offerings.
Point-of-sale (POS) systems provide immediate data on sales transactions, offering a direct measure of consumer demand. This data can be analyzed to identify patterns such as peak shopping hours, popular product combinations, and the impact of promotional activities. By leveraging POS data, businesses can optimize inventory levels, reduce stockouts, and improve customer satisfaction.
Effectively utilizing these real-time data sources requires robust data integration and analysis capabilities. Advanced analytics platforms can aggregate data from social media, web traffic, and POS systems, providing a comprehensive view of current demand signals. Machine learning algorithms can further enhance this process by identifying subtle patterns and correlations that may not be immediately apparent.
Incorporating real-time data into demand forecasting models allows businesses to respond swiftly to market changes, ensuring they remain agile and customer-focused. This proactive approach not only improves the accuracy of demand predictions but also supports better decision-making and resource allocation.
Improved Granularity
Real-time data analytics offers organizations unprecedented granularity in understanding consumer behavior and preferences. This granularity is pivotal for businesses looking to segment their customer base more effectively. By leveraging detailed, real-time data, companies can delve deeper into the nuances of various market segments, leading to enhanced decision-making and strategic planning.
One of the primary types of granular data available through real-time analytics is location-based data. This information allows businesses to pinpoint where their customers are engaging with their products or services. By understanding geographic trends, organizations can tailor their marketing efforts to target specific regions more effectively, ensuring that promotional activities resonate with local audiences.
Demographic information is another critical component of granular data. Real-time analytics can provide insights into age, gender, income levels, and other demographic factors that influence purchasing behavior. This data helps businesses create more personalized marketing campaigns, ensuring that messages and offers are relevant to the specific needs and preferences of different demographic groups.
Transaction history is also a valuable source of granular data. By analyzing past purchase behaviors, businesses can identify patterns and predict future demand with greater accuracy. This insight is crucial for inventory management, as it allows companies to stock products that are likely to be in high demand, reducing the risk of overstocking or stockouts.
The benefits of granular data extend beyond marketing and inventory strategies. With more precise demand forecasts, businesses can optimize their supply chains, enhancing efficiency and reducing costs. Additionally, detailed insights into consumer behavior enable companies to innovate and develop products that better meet the needs of their target market.
In conclusion, real-time data analytics provides the granularity required to understand and predict consumer behavior accurately. By harnessing location-based data, demographic information, and transaction history, businesses can create more targeted marketing campaigns, optimize inventory management, and improve overall demand forecasting. This granular approach is indispensable for companies aiming to stay competitive and responsive in today's dynamic market environment.
Enhanced Responsiveness
In today's fast-paced market environment, the ability to swiftly respond to changes is crucial for business success. Real-time data analytics plays a pivotal role in enhancing this responsiveness by providing continuous monitoring of data streams. This capability allows companies to quickly detect and react to fluctuations in demand, which can be influenced by myriad factors such as competitive actions, economic shifts, or other external variables.
One of the primary advantages of real-time data analytics is the agility it affords organizations. Businesses that can promptly adjust their strategies based on current data are better positioned to capitalize on emerging opportunities or mitigate potential risks. For instance, if a retailer notices a sudden spike in demand for a particular product, they can immediately alter their production schedules to increase supply, ensuring that they meet customer expectations and avoid stockouts. Conversely, if demand drops, they can scale back production to prevent overstock and minimize waste.
Additionally, real-time analytics empowers businesses to launch targeted promotions at the most opportune moments. For example, an e-commerce company might use real-time data to identify when customers are most likely to make purchases, enabling them to deploy effective marketing campaigns that drive sales. Similarly, real-time insights can be used to optimize inventory levels, ensuring that stock is allocated efficiently across different locations to meet regional demand patterns.
Real-world examples underscore the transformative impact of real-time data analytics. A global fashion retailer, for example, leveraged real-time sales data to adjust its inventory levels dynamically across various outlets, significantly reducing excess stock and improving turnover rates. Another case is a leading consumer electronics company that utilized real-time analytics to monitor market trends and swiftly introduce promotional offers, resulting in a substantial increase in sales during peak seasons.
Ultimately, the ability to respond promptly to market changes through real-time data analytics is not just a competitive advantage but a necessity in today's dynamic business landscape. Companies that harness this technology can stay ahead of the curve, making informed decisions that drive growth and enhance customer satisfaction.
Integration with Advanced Technologies
Real-time data analytics, when integrated with advanced technologies like machine learning (ML) and artificial intelligence (AI), has the potential to revolutionize demand forecasting. These technologies can process vast amounts of data at unprecedented speeds, providing insights that traditional analytical methods might overlook. With AI and ML, businesses can identify patterns and trends that are not immediately apparent, leading to more refined and accurate predictions.
For instance, machine learning algorithms can analyze historical sales data, customer behavior, market trends, and even external factors such as weather conditions or economic indicators. By continuously learning from new data, these algorithms can adjust and improve their predictions over time. This dynamic approach allows for a level of precision that static models simply cannot achieve.
Artificial intelligence further enhances this process by automating complex data analysis tasks. AI systems can sift through enormous datasets in real-time, identifying correlations and causations that would take human analysts significantly longer to discover. This capability is particularly beneficial in industries with highly volatile demand patterns, such as retail and e-commerce, where accurate demand forecasting is crucial for inventory management and customer satisfaction.
Several companies have successfully leveraged the integration of AI and ML with real-time data analytics. For example, Amazon uses these technologies to predict product demand and manage its vast inventory efficiently. By analyzing purchasing patterns and customer preferences, Amazon can anticipate which products will be in high demand and adjust its stock levels accordingly, thereby reducing overstock and stockouts.
Similarly, Netflix employs machine learning algorithms to analyze viewing habits and preferences, allowing the company to forecast demand for specific types of content. This data-driven approach enables Netflix to make informed decisions about content acquisition and production, ensuring that they cater to viewer interests effectively.
In conclusion, the integration of real-time data analytics with advanced technologies like AI and ML significantly enhances demand forecasting capabilities. By processing large volumes of data quickly and accurately, these technologies provide businesses with the insights needed to stay competitive in an ever-changing market landscape.
Reduced Forecasting Errors
One of the significant benefits of real-time data analytics is the marked reduction in forecasting errors. Traditional forecasting methods often rely on historical data that may not reflect current market dynamics, leading to discrepancies between predicted and actual demand. Common sources of forecasting errors include outdated data, seasonal fluctuations, sudden market shifts, and human biases in data interpretation.
Real-time data analytics addresses these issues by utilizing up-to-date information, allowing businesses to respond swiftly to changes in the market. This dynamic approach incorporates the latest sales data, customer behavior insights, and external factors such as economic conditions and social trends. By continually updating the data, companies can achieve more accurate and timely demand predictions, thereby reducing the gap between forecasted and actual demand.
Studies have demonstrated the efficacy of real-time data analytics in minimizing forecasting errors. For instance, a report by McKinsey & Company revealed that organizations leveraging real-time analytics saw a 20-30% reduction in forecasting errors compared to those using traditional methods. Another study by Deloitte found that companies employing real-time data analytics experienced a 15% improvement in forecast accuracy, leading to better inventory management and reduced stockouts.
Moreover, by integrating machine learning algorithms, real-time analytics can identify patterns and trends that might be overlooked by human analysts. These advanced algorithms can process vast amounts of data at unprecedented speeds, providing actionable insights that further refine demand forecasting models. Consequently, businesses can optimize their supply chains, reduce excess inventory, and improve overall operational efficiency.
In conclusion, real-time data analytics offers a robust solution to the perennial challenge of forecasting errors. By leveraging up-to-date information and sophisticated analytical tools, businesses can significantly enhance the accuracy of their demand predictions, ultimately leading to improved customer satisfaction and increased profitability.
Cost Efficiency and Resource Optimization
Accurate demand forecasting facilitated by real-time data analytics significantly enhances a business's ability to allocate resources and manage costs effectively. By leveraging real-time data, companies can predict demand with greater precision, allowing them to adjust their inventory levels accordingly. This optimization results in a reduction of excess stock, minimizing waste and associated holding costs.
One of the primary financial benefits of accurate demand forecasting is the reduction in both overstock and stockouts. Overstocking can lead to increased storage costs and potential obsolescence, whereas stockouts can result in lost sales and dissatisfied customers. Real-time data analytics provides businesses with the insights needed to maintain an optimal balance, ensuring that inventory levels are just right to meet customer demand without incurring unnecessary expenses.
Furthermore, improved demand forecasting contributes to more efficient supply chain management. By predicting demand more accurately, businesses can streamline their supply chain operations, reducing lead times and enhancing the overall efficiency of their logistics. This not only lowers transportation and warehousing costs but also improves the agility of the supply chain, enabling companies to respond swiftly to market changes.
Several companies have already demonstrated the financial benefits of implementing real-time data analytics for demand forecasting. For instance, a leading retail chain utilized real-time data to analyze purchasing patterns, leading to a 15% reduction in inventory costs. Similarly, a global manufacturing firm employed real-time analytics to forecast demand more accurately, resulting in a 20% decrease in production-related expenses and a 10% improvement in delivery times.
Through the strategic use of real-time data analytics, businesses can achieve significant cost savings and resource optimization. These benefits contribute to overall business efficiency, enabling companies to remain competitive in today's dynamic market environment.