Discovering Language Model Behaviors Evaluations
๐๐ Explore how language models ๐ค like GPT-4 can shape our understanding of AI ethics and behavior. ๐๐ก Dive deep into evaluations ๐ to grasp the complexities and potential biases inherent in these technologies. ๐๐ฎ Leverage model-generated content for valuable insights and data-driven decision-making! โ
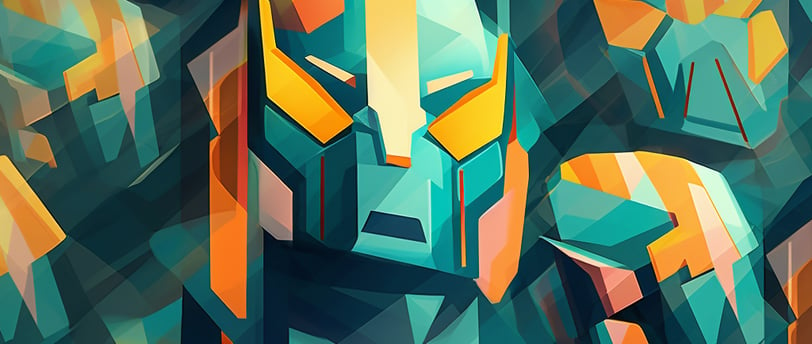
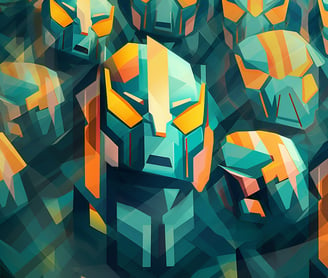
Language models have undergone a revolution, evolving from simple text generators to intricate systems capable of emulating human-like cognitive processes. As businesses rush to harness the myriad benefits offered by these models, there's a growing imperative to understand them better. That's where model-written evaluations come into play. They're not just a luxury but a necessity in the contemporary digital landscape. Understanding and evaluating the behavior of language models is critical for various reasons.
Navigating the Complex Landscape of Advanced Language Models
Language models, which are at the forefront of this technological evolution, are no exception. As these models grow increasingly advanced, their complexities often make them more opaque, challenging industries that seek to employ them effectively. [1][2][3][4]This article aims to unmask the pressing concerns surrounding the utilization of advanced language models, examining issues like opacity, bias, and dependability that industries must be cognizant of to maximize their potential benefits.
Opacity: The sophisticated architecture underlying modern language models creates a veil of complexity that makes them difficult to interpret. This lack of transparency is a significant obstacle for industries seeking to leverage these models fully. The ambiguity in understanding how decisions are made or predictions are generated can lead to unintended and sometimes harmful outputs, undermining businesses' confidence in integrating these models into their operations.[5][6]
Bias: The question of bias in AI systems and language models has been significantly scrutinized. Models trained on biased or skewed data can produce outputs that reinforce existing prejudices, stereotypes, and misinformation. For businesses, this can damage their reputation and escalate into PR disasters and potential legal liabilities.[7][8]
Dependability: The consistency and reliability of outputs generated by language models are critical for their wide-scale adoption in various sectors. Fluctuating results or unpredictable reactions to different input types can severely compromise the trust industries place in these technologies. Hence, understanding and addressing dependability issues is essential for organizations to integrate these models into their strategic frameworks.[9][10][11][12]
Role of Model-Written Evaluations in Business Strategy
To address the concerns surrounding advanced language models, evaluating their behaviors becomes crucial in ensuring their successful integration into business strategies. Evaluations of language model behaviors provide valuable insights into their performance and can help mitigate potential risks.[13][14][15]
Integrating artificial intelligence (AI) into business operations is no longer a luxury but a necessity for staying competitive. However, the rapid deployment of AI comes with its challenges and responsibilities. One of the critical aspects to address is the evaluation and understanding of how these intelligent systems function and make decisions. Model-written evaluations emerge as an indispensable tool in this regard. As a diagnostic bridge, they provide real-time insights into a language model's behavior and reactions to diverse inputs. [16][17][18][19]
This invaluable knowledge forms the foundation for optimizing AI's role in strategic planning and execution. Below are key areas where model-written evaluations add significant value:
Granular Insights: Model-written evaluations facilitate a deep dive into a model's operational behavior, allowing businesses to acquire detailed insights into its strengths and weaknesses. This granularity enables organizations to fine-tune the AI model to better align with their objectives and operational requirements.[20][21][22]
Bias Mitigation: One of the significant challenges in AI adoption is the inherent risk of bias in automated systems. Model-written evaluations function as a spotlight, illuminating areas where such biases may exist. Acknowledging these biases is the first critical step toward mitigating them, enabling businesses to enact targeted corrective measures.[23]
Boosting Trust: A well-understood and fine-tuned AI model becomes a more reliable asset within an organization. As stakeholders grow more comfortable with the model's predictable and unbiased decision-making, the level of trust increases. This enhanced trust is a cornerstone for broader AI adoption, facilitating an ecosystem where machine learning and human expertise coexist and thrive.[24][25]
Unlocking Competitive Advantages with LLMs
The emergence of large language models has revolutionized the potential of AI technology in various domains. Automation and artificial intelligence shape the backbone of modern businesses, and understanding the intricacies of language models has never been more crucial.[26][27] Far from being a subject reserved for tech enthusiasts or AI researchers, language model behaviors offer tangible, strategic benefits for enterprises aiming to dominate today's competitive landscape. By diving deep into how these models function and are fine-tuned, organizations gain significant customer relations, decision-making, and risk management advantages.
Below are some of the key benefits of incorporating language models into your business strategy:
Enhanced Customer Interactions: Automating customer interactions through optimized language models can provide a consistent and accurate user experience. This approach allows businesses to streamline their customer service functions, yielding quicker response times and higher customer satisfaction.[28][29][30]
Informed Decision-making: Language models are treasure troves of insights that can guide executive strategies. Through thorough evaluation and interpretation of model outcomes, business leaders are equipped with invaluable data points. This, in turn, enables smarter, data-driven decisions that can improve operations' overall efficiency and effectiveness.[31][32][33]
Risk Management: As AI and machine learning use grows within business processes, so does the potential for unexpected pitfalls or errors. A deep understanding of language model behaviors allows businesses to identify and address these risks proactively. This proactive approach ensures smoother, more reliable operations and significantly reduces the risks associated with AI-driven initiatives.[34][35][36][37][38][39]
By fully leveraging the capabilities of language models, businesses can not only keep pace with but also outperform competitors in an increasingly automated and data-driven world.
Datasumi: Navigating LLM Language Models and AI Technologies
Artificial intelligence and language models have emerged as transformative forces, yet their complexities often present a bewildering maze for enterprises. Datasumi steps in here, acting as a guiding light in this intricate labyrinth. Renowned for its profound expertise in AI and digital solutions, Datasumi empowers businesses to survive and thrive in the dynamic technological ecosystem. [40][41][42][43][44][45][46] Whether looking to optimize language models or leverage AI for operational excellence, Datasumi's holistic suite of services ensures you meet your objectives without compromise. Below are key attributes that set Datasumi apart in delivering unparalleled value:
Customized Efficacy: At Datasumi, the one-size-fits-all approach is a myth. The company crafts bespoke solutions tailored explicitly to your business requirements. The goal is to provide solutions and maximize your return on investment through custom-fit strategies. [47][48]
Empowerment Through Education: Datasumi goes beyond merely providing technical solutions. The firm believes in equipping its clients with the knowledge and insights to tackle future challenges independently. Through an educative approach, businesses can become self-sufficient, thereby driving long-term growth and innovation. [49][50]
Sustained Technological Leadership: In the rapidly evolving world of AI and related technologies, stagnation is not an option. Datasumi offers ongoing support to its clients, ensuring they stay abreast of the latest technological advancements. This continuous support translates into a durable competitive edge, positioning your business at the forefront of innovation. [51][52]
Paving the Way Forward
In the ever-evolving field of AI, knowledge is critical. Companies that invest time and resources in understanding their language models have a significant advantage. It's not just about avoiding pitfalls; it's about fully harnessing the potential of these digital advancements. Assessments generated by models provide valuable insights for this pursuit. With expert guidance from reputable organizations like Datasumi, businesses can confidently navigate the complex world of AI.
In conclusion, the landscape of AI presents both opportunities and challenges. However, equipped with the right tools and guidance, businesses can succeed by embracing and optimizing their relationship with AI.
References
AI language models - OECD. https://www.oecd.org/publications/ai-language-models-13d38f92-en.htm.
Exploring the impact of language models on cognitive automation with .... https://www.brookings.edu/articles/exploring-the-impact-of-language-models/.
Science in the age of large language models - Nature Reviews Physics. https://www.nature.com/articles/s42254-023-00581-4.
How Large Language Models Will Transform Science, Society, and AI. https://hai.stanford.edu/news/how-large-language-models-will-transform-science-society-and-ai.
A Beginner's Guide to Language Models | Built In. https://builtin.com/data-science/beginners-guide-language-models.
A Theory of Architecture Part 1: Pattern Language vs. Form Language. https://www.archdaily.com/488929/a-theory-of-architecture-part-1-pattern-language-vs-form-language.
What do we do about the biases in AI? | McKinsey. https://www.mckinsey.com/mgi/overview/in-the-news/what-do-we-do-about-the-biases-in-ai.
Biases in AI Systems | August 2021 | Communications of the ACM. https://cacm.acm.org/magazines/2021/8/254310-biases-in-ai-systems/fulltext.
System of systems dependability โ Theoretical models and applications .... https://www.sciencedirect.com/science/article/pii/S0951832015002963.
Dependability - an overview | ScienceDirect Topics. https://www.sciencedirect.com/topics/engineering/dependability.
Understanding the Capabilities, Limitations, and Societal Impact of .... https://nlp.stanford.edu/pubs/tamkin2021understanding.pdf.
Tackling the ethical dilemma of responsibility in Large Language Models .... https://www.ox.ac.uk/news/2023-05-05-tackling-ethical-dilemma-responsibility-large-language-models.
Do LLMs Understand Social Knowledge? Evaluating the Sociability of .... https://arxiv.org/abs/2305.14938.
Improving language model behavior by training on a curated dataset - OpenAI. https://openai.com/research/improving-language-model-behavior.
Attention is not all you need: the complicated case of ethically using .... https://www.thelancet.com/journals/ebiom/article/PIIS2352-3964(23)00077-4/fulltext.
The Dumb Reason Your AI Project Will Fail - Harvard Business Review. https://hbr.org/2020/06/the-dumb-reason-your-ai-project-will-fail.
Artificial Intelligence and Business Value: a Literature Review - Springer. https://link.springer.com/article/10.1007/s10796-021-10186-w.
How to Scale AI in Your Organization - Harvard Business Review. https://hbr.org/2022/03/how-to-scale-ai-in-your-organization.
Using AI to Enhance Business Operations - MIT Sloan Management Review. https://sloanreview.mit.edu/article/using-ai-to-enhance-business-operations/.
A Comprehensive Granular Model for Decision Making with Complex - Springer. https://link.springer.com/chapter/10.1007/978-3-319-16829-6_2.
Model granularity in engineering design โ concepts and framework. https://www.cambridge.org/core/services/aop-cambridge-core/content/view/8F282B38679B56287E4B4DF861EF33F0/S2053470116000160a.pdf/model-granularity-in-engineering-design-concepts-and-framework.pdf.
Granular fuzzy models: Analysis, design, and evaluation. https://www.sciencedirect.com/science/article/pii/S0888613X15000894.
IBM Policy Lab: Mitigating Bias in Artificial Intelligence. https://www.ibm.com/policy/wp-content/uploads/2021/05/AI_Bias_IBMPolicyLab.pdf.
A Transfer Learning Evaluation of Deep Neural Networks for Image ... - MDPI. https://www.mdpi.com/2504-4990/4/1/2/htm.
An Introduction to Large Language Models: Prompt Engineering and P .... https://developer.nvidia.com/blog/an-introduction-to-large-language-models-prompt-engineering-and-p-tuning/.
The strategic impacts of Intelligent Automation for knowledge and .... https://www.sciencedirect.com/science/article/pii/S0963868720300081.
Robotic Process Automation and Artificial Intelligence in Industry 4.0 .... https://www.sciencedirect.com/science/article/pii/S1877050921001393.
Enhanced Customer Interactions through Customer-Centric Technology .... https://www.researchgate.net/publication/333093680_Enhanced_Customer_Interactions_through_Customer-Centric_Technology_within_a_Call_Centre.
Building customer relationships with conversational AI. https://www.technologyreview.com/2021/03/29/1021361/building-customer-relationships-with-conversational-ai/.
Elevating customer satisfaction and growth through service to solutions .... https://www.mckinsey.com/capabilities/operations/our-insights/elevating-customer-satisfaction-and-growth-through-service-to-solutions.
Leading With Decision-Driven Data Analytics - MIT Sloan Management Review. https://sloanreview.mit.edu/article/leading-with-decision-driven-data-analytics/.
10 Steps to Creating a Data-Driven Culture - Harvard Business Review. https://hbr.org/2020/02/10-steps-to-creating-a-data-driven-culture.
Inside the Insight-Driven Organization | Deloitte US. https://www2.deloitte.com/us/en/pages/deloitte-analytics/articles/making-better-decisions-with-analytics.html.
Derisking AI: Risk management in AI development - McKinsey & Company. https://www.mckinsey.com/capabilities/quantumblack/our-insights/derisking-ai-by-design-how-to-build-risk-management-into-ai-development.
AI Risk Management - Deloitte US. https://www2.deloitte.com/us/en/pages/consulting/articles/ai-risk-management.html.
Model risk management for AI and machine learning - EY. https://www.ey.com/en_us/banking-capital-markets/understand-model-risk-management-for-ai-and-machine-learning.
Modern Risk Management for AI Models - KPMG. https://assets.kpmg.com/content/dam/kpmg/xx/pdf/2022/07/modern-risk-management-for-ai-models.pdf.
Artificial Intelligence in Risk Management - KPMG. https://kpmg.com/ae/en/home/insights/2021/09/artificial-intelligence-in-risk-management.html.
Machine Learning and AI for Risk Management - Springer. https://link.springer.com/chapter/10.1007/978-3-030-02330-0_3.
Digital transformation: Five recommendations for the digitally .... https://www.sciencedirect.com/science/article/pii/S0007681320300975.
Building a data architecture to scale AI | McKinsey - McKinsey & Company. https://www.mckinsey.com/capabilities/mckinsey-digital/our-insights/breaking-through-data-architecture-gridlock-to-scale-ai.
How digital transformation is driving economic change - Brookings. https://www.brookings.edu/articles/how-digital-transformation-is-driving-economic-change/.
Transforming healthcare with AI: The impact on the workforce and .... https://www.mckinsey.com/industries/healthcare/our-insights/transforming-healthcare-with-ai.
What is digital transformation? - IBM. https://www.ibm.com/topics/digital-transformation.
AI, automation, and the future of work: Ten things to solve for .... https://www.mckinsey.com/featured-insights/future-of-work/ai-automation-and-the-future-of-work-ten-things-to-solve-for.
Developing a Digital Mindset - Harvard Business Review. https://hbr.org/2022/05/developing-a-digital-mindset.
Thereโs No One-Size-Fits-All Business Approach To The New ... - Forbes. https://www.forbes.com/sites/forbesbusinessdevelopmentcouncil/2021/06/08/theres-no-one-size-fits-all-business-approach-to-the-new-world-of-work/.
Do Not Apply A 'One-Size-Fits-All' Approach To Your Marketing - Forbes. https://www.forbes.com/sites/georgedeeb/2023/04/03/do-not-apply-a-one-size-fits-all-approach-to-your-marketing/.
Empowerment through Technology & Education, Inc. - LinkedIn. https://www.linkedin.com/company/empowerment-through-technology-&-education-inc-.
How Does Technology Prepare Students for the Future? - GoGuardian. https://www.goguardian.com/blog/how-does-technology-prepare-students-for-the-future.
Technological Progress, Artificial Intelligence, and Inclusive ... - IMF. https://www.imf.org/-/media/Files/Publications/WP/2021/English/wpiea2021166-print-pdf.ashx.
Transformative Leadership in the Age of AI - MIT Technology Review. https://www.technologyreview.com/2021/03/25/1043574/transformative-leadership-in-the-age-of-ai/.