Mistral's New Fine-Tuning Tools: Customize AI Models Faster
Discover Mistral's latest innovation in AI - fine-tuning tools that simplify and accelerate the customization of large language models. Achieve more accurate and valuable outputs for your enterprise, without the hefty price tag.
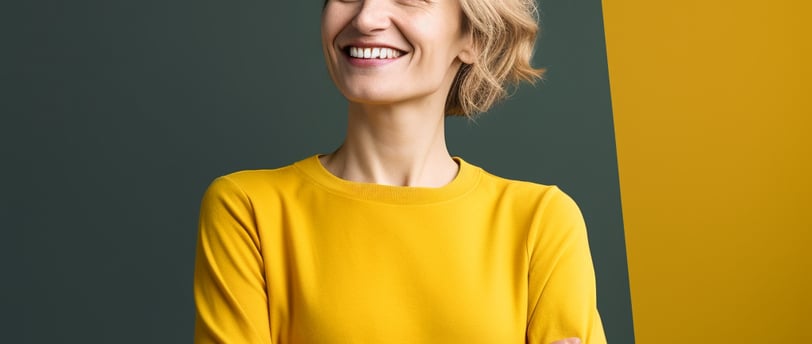
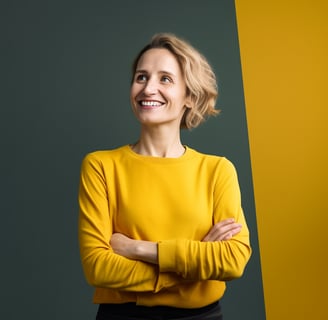
Mistral, a leading company in the field of artificial intelligence, has recently introduced new fine-tuning tools designed to simplify and expedite the process of customizing its models. These tools are aimed at enhancing the output of large language models (LLMs) and tailoring them to meet specific enterprise requirements.
The significance of fine-tuning lies in its ability to improve the accuracy and relevance of model responses. By fine-tuning, organizations can extract more value and precision from their generative AI applications, ensuring they meet their unique business needs.
However, the process of fine-tuning is not without its challenges. It can be a costly endeavor, which may deter some enterprises from leveraging this beneficial process. Mistral's new fine-tuning tools are set to address this issue, making it more accessible and affordable for businesses to fine-tune their AI models and achieve optimal results.
Introduction to Mistral's Fine-Tuning Tools
Mistral has recently launched a new suite of fine-tuning tools designed to revolutionize the customization of large language models (LLMs). This significant development addresses the growing need for more precise and tailored outputs from these models, especially in enterprise settings where specificity and accuracy are paramount. Fine-tuning, a process that involves adjusting a pre-trained model's parameters using a smaller, domain-specific dataset, is critical for enhancing the performance and relevance of LLMs in various applications.
Fine-tuning allows organizations to leverage the robust capabilities of LLMs while adapting them to meet unique and specific requirements. By refining the model's responses, companies can achieve higher accuracy in tasks such as customer support, content generation, and data analysis. Traditionally, this process has been complex and time-consuming, often requiring substantial computational resources and expertise in machine learning.
The introduction of Mistral's fine-tuning tools marks a significant leap forward in simplifying and accelerating this process. These tools are engineered to streamline customization, enabling enterprises to modify their LLMs with greater efficiency and less technical overhead. By reducing the barriers to fine-tuning, Mistral empowers organizations to enhance their LLM outputs more effectively, ensuring that the models are better aligned with their specific operational needs.
Moreover, the new tools offer an intuitive interface and advanced features that cater to both novice and experienced users. This user-friendly approach democratizes the fine-tuning process, making it accessible to a broader range of professionals within an organization. As a result, companies can now achieve optimal model performance with reduced time and effort, fostering innovation and productivity while maintaining high standards of output quality.
In the rapidly evolving landscape of generative AI, enterprises are continually seeking ways to enhance the precision and utility of their models. Fine-tuning has emerged as a pivotal process in this endeavor, offering a means to tailor pre-trained models to meet specific organizational requirements. By refining a model's parameters based on domain-specific data, fine-tuning enables enterprises to achieve more accurate and contextually relevant responses, thereby maximizing the value derived from their AI applications.
One of the primary advantages of fine-tuning for enterprises is the ability to address unique business challenges with greater specificity. For instance, a financial institution may leverage fine-tuning to enhance its risk assessment models, ensuring that the AI can accurately parse complex financial data and provide nuanced insights. Similarly, a healthcare provider might fine-tune a model to better understand medical terminology and patient records, leading to improved diagnostic capabilities and personalized treatment recommendations.
Moreover, fine-tuning can significantly reduce the amount of manual intervention required to rectify inaccuracies or irrelevant outputs. In customer service applications, for example, a fine-tuned model can more effectively interpret and respond to customer inquiries, reducing the need for human agents to step in and correct errors. This not only streamlines operations but also enhances customer satisfaction by providing faster and more reliable service.
Another critical benefit of fine-tuning is the ability to maintain and improve model performance over time. As enterprises accumulate more data, fine-tuning allows for continuous model updates, ensuring that the AI remains aligned with evolving business needs and industry standards. This iterative improvement process is essential for maintaining competitive advantage and operational efficiency in a dynamic market environment.
In essence, fine-tuning transforms generic models into specialized tools tailored to an enterprise's unique requirements. By facilitating more accurate and useful model responses, fine-tuning empowers organizations to harness the full potential of their generative AI applications, driving innovation and operational excellence across various sectors.
Challenges of Traditional Fine-Tuning Methods
Traditional fine-tuning methods for large language models (LLMs) have long been associated with a variety of challenges, primarily due to their high costs and resource-intensive nature. These methods often require substantial computational power and extensive data sets, which can be prohibitive for many enterprises. The financial and logistical barriers to entry mean that only the most well-resourced organizations can fully leverage the potential of LLM customization.
One of the most significant challenges is the sheer amount of computational resources needed. Fine-tuning a large language model typically requires specialized hardware, such as GPUs or TPUs, and considerable energy consumption. This not only increases operational costs but also contributes to a larger carbon footprint, raising sustainability concerns. Smaller enterprises or startups, which may not have access to such resources, often find themselves at a disadvantage.
Additionally, the process demands extensive datasets to train the model effectively. Compiling, cleaning, and labeling these datasets can be a time-consuming and labor-intensive task. Companies often have to invest in acquiring proprietary data or rely on third-party data providers, further escalating costs. Moreover, the expertise required to manage and execute these fine-tuning processes is another significant hurdle. Skilled data scientists and machine learning engineers are in high demand, making it challenging for smaller organizations to attract and retain such talent.
Real-world examples illustrate these challenges vividly. For instance, a mid-sized e-commerce company aiming to fine-tune an LLM for personalized customer interactions might find the costs and resource requirements overwhelming. The need for continuous updates to the model, in response to changing market trends and consumer behaviors, exacerbates these issues. As a result, many enterprises are unable to fully exploit the benefits of LLM customization, leaving them at a competitive disadvantage.
These traditional fine-tuning methods, while powerful, are not always accessible or practical for every organization. The high costs, resource demands, and expertise required create significant barriers, highlighting the need for more efficient and accessible solutions in the realm of LLM customization.
Fine-tuning machine learning models has historically been a complex process, demanding significant expertise and computational resources. Mistral's newly launched fine-tuning tools aim to alleviate these challenges, making the customization of models not only easier but also faster and more cost-effective.
Streamlined Interface
One of the standout features of Mistral's fine-tuning tools is the streamlined interface. The user-friendly design ensures that even those with limited technical knowledge can navigate the fine-tuning process with ease. The interface simplifies complex configurations, offering intuitive options and guided steps to help users achieve optimal results. This democratization of fine-tuning opens doors for a wider range of users, from data scientists to business analysts, allowing them to harness the power of customized models without needing deep technical expertise.
Automated Hyperparameter Tuning
Another significant advancement is the automated hyperparameter tuning feature. Traditionally, selecting the right hyperparameters required extensive experimentation and domain knowledge. Mistral's tools incorporate advanced algorithms that automatically adjust hyperparameters based on the specific dataset and desired outcomes. This automation not only accelerates the fine-tuning process but also enhances model performance by identifying the most effective configurations.
Scalability and Efficiency
Mistral's fine-tuning tools are designed with scalability in mind. The tools leverage cloud-based infrastructure, allowing users to scale their fine-tuning efforts according to their needs. Whether fine-tuning a single model or multiple models simultaneously, the tools ensure efficient use of computational resources, reducing both time and cost. This scalability is particularly beneficial for enterprises looking to deploy customized models across various applications and departments.
Real-Time Feedback and Monitoring
The inclusion of real-time feedback and monitoring capabilities further enhances the user experience. Users can track the progress of the fine-tuning process in real-time, enabling them to make informed decisions and adjustments on the fly. Detailed metrics and visualizations provide insights into model performance, helping users to quickly identify and address any issues that may arise.
Overall, Mistral's new fine-tuning tools represent a significant leap forward in making model customization more accessible, efficient, and cost-effective. By addressing the traditional challenges associated with fine-tuning, these tools empower users to create highly tailored machine learning models with greater ease and precision.
Benefits of Using Mistral's Fine-Tuning Tools
Mistral's fine-tuning tools offer a plethora of advantages for enterprises looking to enhance their machine learning models. One of the primary benefits is the acceleration of deployment times. By streamlining the customization process, Mistralโs tools allow organizations to quickly adapt models to specific tasks, thereby reducing the time from development to deployment. This efficiency is critical in industries where time-to-market can significantly influence competitiveness.
Cost reduction is another substantial benefit. Traditional model customization often requires extensive computational resources and expert intervention, both of which can be expensive. Mistralโs fine-tuning tools, however, are designed to minimize these requirements by providing a more efficient and user-friendly interface. This not only reduces the direct costs associated with computational power but also decreases the need for specialized personnel, thus lowering overall operational expenses.
Moreover, Mistral's tools enable more precise model customization. Enterprises can fine-tune models to meet their specific needs with greater accuracy, ensuring that the outputs are highly relevant and tailored to their unique data sets and objectives. This precision leads to better performance in real-world applications, enhancing the overall effectiveness of the deployed models.
To illustrate these benefits, consider a hypothetical scenario where a retail company uses Mistralโs fine-tuning tools to customize a recommendation engine. The tools allow the company to quickly adjust the model to account for seasonal trends and individual customer preferences, leading to a more personalized shopping experience. This rapid and precise customization helps the company increase sales and customer satisfaction.
Another example is in the healthcare industry, where a medical research institution leverages Mistralโs tools to fine-tune a diagnostic model. The institution can efficiently tailor the model to recognize specific patterns in patient data, resulting in faster and more accurate diagnoses. This not only improves patient outcomes but also optimizes the institutionโs resource allocation.
In summary, Mistral's fine-tuning tools provide significant advantages by enabling faster deployment, reducing costs, and allowing for more precise model customization. These benefits are crucial for enterprises aiming to stay competitive and maximize the value derived from their machine learning initiatives.
Conclusion and Future Implications
Mistral's introduction of fine-tuning tools represents a significant advancement in the field of artificial intelligence, particularly in the customization of language models. These tools streamline the process of adapting AI models to specific tasks, making it more accessible and efficient for enterprises to deploy tailored solutions. The enhanced capability to fine-tune models quickly and effectively can lead to more precise and relevant outputs, thereby increasing the overall utility and application of AI in various industries.
One of the most profound impacts of these tools is their potential to democratize AI by lowering the technical barriers to entry. Organizations that previously lacked the resources to develop and customize sophisticated models can now leverage Mistral's tools to create bespoke solutions tailored to their unique needs. This could lead to a surge in innovative applications across diverse sectors, from healthcare and finance to customer service and beyond.
Looking ahead, the implications for the AI industry are substantial. As these fine-tuning tools become more widespread, we can expect a proliferation of highly specialized, efficient AI models. This trend will likely accelerate the adoption of AI technologies in enterprise environments, as businesses increasingly recognize the value of customized AI solutions. Furthermore, ongoing advancements and refinements in these tools could enhance their capabilities, offering even greater precision and efficiency in model customization.
Future developments may also focus on improving the user experience, making these tools even more intuitive and accessible to a broader audience. Enhancements in AI and LLM fine-tuning, supported by robust and user-friendly tools, could ultimately lead to more seamless integration of AI into everyday business processes. This would not only drive operational efficiencies but also open up new possibilities for innovation and growth across various industries.
In summary, Mistral's fine-tuning tools mark a pivotal step forward in the evolution of AI customization. By enabling faster and easier model adaptation, these tools stand to significantly impact the way enterprises utilize AI, fostering a future where tailored, high-performance AI solutions are within reach for all.
Can Mistral's tools be integrated with existing AI platforms?
Mistral's AI models and tools can be integrated with existing AI platforms and services, as evidenced by the search results:
Integration with Microsoft Azure AI
Mistral has partnered with Microsoft to integrate their models into the Azure AI platform[2]:
Mistral's flagship model, Mistral Large, is available on Azure AI and can be accessed through Azure AI Studio.
The smaller Mistral Small model is also available in the Azure AI model catalog and can be deployed as a service through Azure AI[5].
This integration allows Azure customers to leverage Mistral's models through Azure's AI services, tools, and infrastructure.
Integration with Databricks
Mistral has partnered with Databricks to integrate their models into the Databricks Data Intelligence Platform[3]:
Mistral's models are available in the Databricks Marketplace and can be accessed natively within the Databricks platform.
Customers can interact with Mistral models through the Mosaic AI Playground, use them as model endpoints, and customize them using their own data.
This integration enables Databricks customers to leverage Mistral's models for generative AI applications while benefiting from Databricks' data governance and security features.
Integration through Appy Pie Connect
The search results mention that Mistral AI can be integrated with other applications and services using Appy Pie Connect, an AI-driven integration platform[1]. This allows businesses to automate workflows and processes by connecting Mistral AI with other tools they use.
In summary, Mistral has established partnerships and integrations with major AI platforms like Microsoft Azure and Databricks, allowing their models to be seamlessly utilized within these existing ecosystems. Additionally, integration platforms like Appy Pie Connect facilitate connecting Mistral AI with other business applications and services[1].
Citations
Mistral AI. (2024, June 5). Mistral launches fine-tuning tools to make customizing its models easier and faster. VentureBeat. https://venturebeat.com/ai/mistral-launches-fine-tuning-tools-to-make-customizing-its-models-easier-and-faster/Mistral
Diaflow. (2024, March 30). What is Mistral AI? https://www.diaflow.io/blog/what-is-mistral-ai
Toolify AI. (2024, June 6). Understanding the Impact of Fine-tuning on Performance. https://www.toolify.ai/ai-news/understanding-the-impact-of-finetuning-on-performance-481808Fine-tuning Mistral 7B with the Samantha dataset adds Samantha's personality, making it a helpful, friendly virtual assistant. However, fine-tuning can impact the original model's performance. Aligning models for best results is crucial.
Microsoft. (2024, June 6). Introducing Mistral Small: Empowering Developers with Efficient LLMs on Azure AI Models as a Service. https://techcommunity.microsoft.com/t5/ai-machine-learning-blog/introducing-mistral-small-empowering-developers-with-efficient/ba-p/4127678
Microsoft. (2023, November). Microsoft and Mistral AI announce new partnership to accelerate AI innovation and introduce Mistral Large first on Azure. https://azure.microsoft.com/en-us/blog/microsoft-and-mistral-ai-announce-new-partnership-to-accelerate-ai-innovation-and-introduce-mistral-large-first-on-azure/
Microsoft announced a multi-year partnership with Mistral AI, introducing Mistral Large, Mistral AI's flagship commercial model, first on Azure AI. The partnership focuses on supercomputing infrastructure, scaling to market, and AI research and development.
Databricks. (2024). Databricks invests in Mistral AI and integrates Mistral AI's models into Databricks Data Intelligence. https://www.databricks.com/blog/databricks-invests-mistral-ai-and-integrates-mistral-ais-models-databricks-data-intelligence
WORK SMARTER WITH DATASUMI
Products & Services
Contact Us โ๏ธ
About us
Terms of Service ๐
GDPR Statement ๐ช๐บ
Learn about
Privacy Policy ๐
Our Blog ๐